In an industry where a single miscalculation can impact millions of lives, pharmaceutical companies are witnessing an unprecedented transformation through AI agents – autonomous systems that can process decades of research in hours and predict drug interactions with remarkable accuracy.
These AI agents, capable of learning, reasoning, and performing tasks that once required human intelligence, are not just enhancing operational efficiencies but are also pushing the boundaries of what’s possible in medical research and treatment. However, as these autonomous systems delve deeper into critical decision-making processes, the cornerstone of their integration into the pharma sector hinges on one fundamental attribute: trustworthiness.
For pharmaceutical companies, the journey towards embedding AI into their core operations isn’t merely about technological adoption; it’s about cultivating an unwavering trust in these digital entities. Trust in AI agents is indispensable, not just for compliance with stringent regulatory frameworks like the FDA’s guidelines, but also for maintaining the ethical standards that underpin healthcare. This trust is the linchpin that ensures AI’s potential is harnessed to improve patient outcomes, streamline workflows, and ultimately, foster innovation while upholding the integrity and safety that patients and healthcare professionals expect.
As we navigate this new era, building trustworthy AI agents becomes not just a strategic imperative but a moral one, ensuring that the promise of AI in pharmaceuticals translates into tangible, reliable benefits for all stakeholders involved.
Understanding AI Agents in Pharma
AI agents are intelligent software systems designed to work autonomously or semi-autonomously across dynamic environments. Unlike traditional AI, which is often limited to static automation or data analysis, AI agents possess the ability to independently plan, execute, and optimize tasks in real-time. These systems act as virtual collaborators, seamlessly interacting with existing tools, datasets, and human teams to enhance efficiency and innovation.
AI agents combine generative AI capabilities with natural language processing (NLP), machine learning, and decision-making algorithms to perform tasks such as:
● Analyzing Data: They process vast, unstructured datasets, like prescribing patterns, market trends, and patient demographics.
● Executing Tasks: AI agents autonomously design and implement workflows, whether it’s crafting a marketing campaign or optimizing sales operations.
● Adapting Strategies: These systems continuously learn from feedback, refining their actions to improve outcomes.
For instance, in sales, AI agents can act as virtual sales assistants, equipping representatives with dynamic insights before meetings with HCPs. By integrating data from CRM systems, market research, and sales performance analytics, these agents can provide reps with tailored talking points, anticipate potential objections, and recommend next-best actions to improve conversions. Additionally, agents can streamline routine administrative tasks, such as logging sales calls and updating CRM entries, allowing sales teams to dedicate more time to customer interactions.
Beyond HCP engagement, AI agents can support omnichannel marketing by orchestrating campaigns across digital platforms, such as social media, email, and patient portals. For example, an agent could monitor patient sentiment on social media regarding a specific drug, identify emerging trends, and recommend adjustments to marketing strategies to address concerns or boost awareness. Agents can also optimize ad spend by analyzing campaign performance across channels and reallocating budgets in real-time to maximize ROI.
In market access and pricing, AI agents can analyze payer data and health economics information to recommend optimal pricing strategies that balance profitability with affordability. These systems can also assist in creating tailored value propositions for payers by simulating the impact of pricing strategies on patient access and overall healthcare savings.
Moreover, AI agents can enhance patient engagement in commercial settings. They can personalize educational materials, recommend adherence programs based on individual patient needs, and even facilitate virtual interactions between patients and pharma representatives.
For example, an agent could guide patients through enrollment in support programs, answer queries about medication usage, and provide reminders for prescription refills, fostering loyalty and improving patient outcomes.
By leveraging the versatility of generative AI agents, pharmaceutical companies can not only strengthen engagement with HCPs, payers, and patients but also achieve greater efficiency and scalability in their commercial operations. This shift to AI-powered, data-driven strategies positions pharma to remain competitive in an increasingly complex and fast-evolving market landscape.
The Importance of Trust in AI
In the pharmaceutical sector, where the stakes are life-altering, trust in AI agents is not merely a desirable attribute but a cornerstone for their successful integration. Trustworthiness in AI hinges on several critical elements: accuracy, transparency, security, and ethical considerations.
Firstly, the accuracy of AI agents is paramount; It ensures that AI agents generate reliable and precise outputs, whether in predicting drug interactions, analyzing clinical trial data, or personalizing patient care.
Transparency involves making the decision-making processes of AI agents understandable to human stakeholders, providing clear documentation and audit trails that facilitate oversight and accountability.
Security is another pillar, given the sensitive nature of healthcare data; robust measures must be in place to protect patient information from breaches and ensure compliance with regulations like GDPR and HIPAA.
Ethical considerations are vital, encompassing the mitigation of biases in AI algorithms, ensuring fairness, and adhering to the highest standards of ethical conduct in healthcare.
The impact of establishing trust in AI on businesses is profound. Trust directly influences patient outcomes by ensuring that AI-driven decisions are reliable, leading to safer and more effective treatments.
Stakeholder confidence is bolstered when AI agents are trusted, encouraging investment, fostering partnerships, and accelerating the pace of innovation. Moreover, trust in AI can enhance the company’s reputation, attract top talent, and reassure investors about the long-term viability of AI initiatives in the pharmaceutical sector.
In essence, trust is the linchpin that not only supports the operational integrity of AI in pharma but also catalyzes the industry’s evolution towards a future where AI-driven healthcare improvements are universally accepted and embraced.
Strategies for Building Trustworthy AI Agents
Cultivating trust in AI agents within the pharmaceutical industry is paramount, and this trust hinges on transparency, fairness, robustness, and privacy. To achieve this, organizations must focus on designing AI agents with the following core principles and strategies:
1. Fostering Autonomy with Rigorous Oversight
AI agents in pharma must operate autonomously to perform complex tasks such as drug discovery, clinical trial optimization, and patient monitoring. However, this autonomy must be paired with robust oversight mechanisms to maintain accountability and ensure adherence to regulatory standards.
● Strategy: Implement human-in-the-loop (HITL) systems where critical decisions—such as patient safety protocols or regulatory compliance—are reviewed by experts before execution.
● Example: In clinical trials, autonomous AI agents can analyze patient data to flag adverse events, but final decisions on trial adjustments are overseen by human researchers.
2. Enhancing Perception with Cross-Validated Data Sources
For AI agents to accurately perceive their environment, they must rely on high-quality, diverse, and validated data. In pharma, this involves integrating data from sensors, electronic health records (EHRs), lab results, and real-world evidence (RWE).
● Strategy: Establish comprehensive data pipelines that ensure data integrity, accuracy, and completeness. Utilize multi-modal data sources to give AI agents a holistic perspective in their decision-making.
● Example: A pharmacovigilance AI agent can analyze data from wearable devices, patient-reported outcomes, and clinical reports to predict adverse drug reactions in real time.
3. Rigorous and Transparent Decision-Making Frameworks
Trustworthy AI agents must employ decision-making processes that are explainable, consistent, and aligned with ethical guidelines. This is especially critical in pharma, where decisions impact patient lives and public health.
● Strategy: Develop explainable AI (XAI) models to ensure that the reasoning behind every decision is traceable and understandable by both regulators and healthcare professionals.
● Example: AI agents used in drug approval processes can provide detailed justifications for why certain compounds are prioritized, ensuring transparency for regulatory bodies like the FDA or EMA.
4. Continuous Learning in a Controlled Environment
AI agents in pharma must learn from past experiences to refine their performance. However, this learning must happen within a controlled environment to prevent unintended consequences.
● Strategy: Use reinforcement learning (RL) frameworks with safe exploration techniques to ensure that AI agents only optimize within predefined ethical and regulatory boundaries. Regular audits and retraining on updated datasets can mitigate risks associated with outdated knowledge.
● Example: AI agents used in personalized medicine can refine treatment recommendations over time by analyzing patient responses, but strict guardrails ensure they do not deviate from approved protocols.
5. Building Ethical and Regulatory Compliance into the Design
To earn trust, AI agents must operate within the framework of ethical guidelines and regulatory standards specific to the pharmaceutical industry.
● Strategy: Integrate compliance checks and ethical guidelines directly into AI workflows, ensuring that decisions align with patient safety, data privacy (e.g., HIPAA, GDPR), and equitable treatment practices.
● Example: AI agents used for patient recruitment in clinical trials can be programmed to ensure demographic diversity and avoid biases, thereby adhering to ethical inclusion standards.
6. Collaborating with Stakeholders for Validation and Trust
Building trust requires collaboration between AI developers, pharmaceutical experts, regulators, and end-users (e.g., doctors and patients).
● Strategy: Involve key stakeholders in the design, validation, and deployment phases of AI agents to ensure alignment with real-world needs and expectations.
● Example: Co-develop AI solutions with input from regulatory authorities, ensuring they meet approval criteria while addressing industry-specific challenges like drug safety monitoring.
By embedding these strategies into the lifecycle of AI agent development, pharmaceutical companies can ensure that their AI systems are not only autonomous and capable but also trustworthy, ethical, and aligned with the industry’s highest standards.
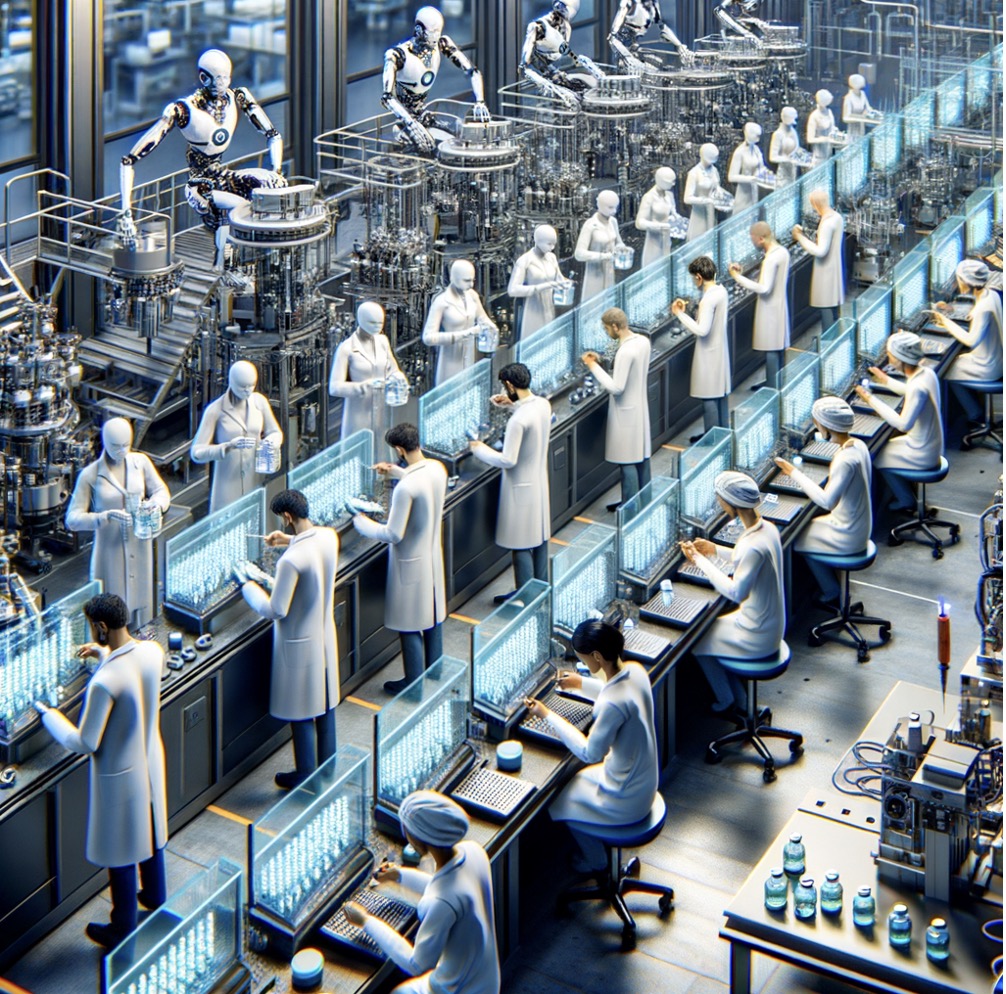
Benefits of AI Agents
AI Agents Enhance Business Efficiency
AI agents are transforming traditional workflows into agile, data-driven processes, reshaping operational landscapes across industries. From simple rule-based systems to advanced machine learning algorithms, these agents bring unprecedented efficiency through autonomous learning and adaptive capabilities. By analyzing outcomes from past decisions, they refine processes, driving breakthroughs in drug discovery, optimizing clinical trial protocols, and streamlining supply chain logistics.
In the pharmaceutical industry, the integration of AI agents is revolutionizing workforce efficiency. By automating routine tasks such as data entry, compliance checks, and preliminary data analysis, these systems dramatically reduce human error and free up valuable resources. This automation enables personnel to focus on high-value, strategic activities like complex problem-solving, innovative drug discovery, and patient-centric product development, fostering innovation and competitive advantage.
AI agents excel in orchestrating and analyzing complex datasets, offering a holistic view of operations. In healthcare, for instance, they manage patient records, clinical trial data, and real-time supply chain analytics to ensure seamless resource allocation. Similarly, in the defense sector, these technologies optimize logistics and strategic planning, analyzing vast data sets to anticipate and address resource needs effectively.
Beyond operational improvements, AI agents significantly enhance collaboration within organizations. Integrated with platforms like Slack or Microsoft Teams, they facilitate real-time information sharing and decision-making. By providing seamless access to knowledge bases and AI-driven insights, they empower teams to consult expertise quickly and efficiently. For example, Novartis has leveraged AI agents to improve internal communication and knowledge sharing, resulting in faster decision-making processes and heightened productivity.
A growing trend is the development of customized AI agents tailored to specific organizational needs. These proprietary systems go beyond general automation, learning from company-specific data to deliver bespoke solutions aligned with strategic goals. Such tailored AI not only automates workflows but also evolves to address unique business challenges, offering a competitive edge that is difficult for rivals to replicate. This bespoke approach ensures that internal processes are optimized to support innovation and sustained market leadership.
AI Agents Are Highly Scalable
The scalability of AI agents provides an unmatched advantage, particularly during peak operational periods like drug launches or regulatory submissions, where the demand for analysis, compliance checks, and real-time monitoring surges. AI agents can handle these fluctuations without the need for additional human resources, ensuring that the company can respond promptly and effectively.
The scalability of AI agents allows both startups and large enterprises to adapt their operations dynamically. Startups can leverage AI agents to compete with larger entities by automating key processes without the proportional increase in overheads. Conversely, large enterprises can use AI agents to manage complex, global operations more efficiently. This democratization of AI technology ensures that innovation is not confined to those with vast resources, leveling the playing field and fostering a more competitive and innovative pharmaceutical landscape.
AI Agents Have Real Time Decision Making
In the pharmaceutical value chain, real-time decision-making enabled by AI agents is a game-changer, driving agility, precision, and responsiveness across critical operations. These agents continuously process and analyze vast, dynamic datasets from sources like clinical trial systems, electronic health records (EHRs), wearable devices, manufacturing sensors, and supply chains, delivering actionable insights instantaneously.
For instance, In clinical trials, AI agents monitor patient data in real time, flagging adverse events or deviations from protocols to allow immediate intervention, thereby safeguarding patient safety and improving trial outcomes.
Similarly, in manufacturing, AI systems detect anomalies such as equipment malfunctions or deviations in product quality, enabling automatic adjustments or preventive maintenance to avoid costly downtime and ensure regulatory compliance.
In supply chain management, AI agents analyze live data on inventory levels, transportation logistics, and market demand to reroute shipments or optimize inventory distribution in response to disruptions or demand spikes, ensuring timely delivery of life-saving medicines.
Moreover, AI-powered commercial systems dynamically adapt marketing and sales strategies based on real-time market trends, customer behavior, and competitive intelligence, maximizing ROI and market impact.
These capabilities are particularly invaluable in scenarios like pandemics or drug recalls, where rapid, data-driven decisions can mitigate risks, reduce costs, and save lives.
By embedding real-time decision-making into the pharma ecosystem, companies can enhance operational efficiency, ensure patient-centric care, and maintain a competitive edge in an ever-evolving industry.
AI Agents Are Adaptable and Learn
AI agents exhibit iterative learning and adaptation, continuously refining their processes based on new data and improving over time. This iterative learning is crucial in pharmaceutical R&D, where AI can analyze clinical trial data to suggest modifications that could enhance trial outcomes.
For example, in market access strategies, these agents dynamically model pricing and reimbursement scenarios by analyzing diverse datasets such as regional healthcare budgets, competitor pricing, and patient affordability metrics. This adaptability helps companies tailor market entry strategies to maximize product uptake in different geographies.
In medical affairs, AI agents learn from real-time interactions with HCPs and scientific data, personalizing medical content dissemination to align with the specific interests or knowledge gaps of HCPs, enhancing engagement and educational impact.
Additionally, AI agents are revolutionizing post-market surveillance by continuously analyzing patient feedback, adverse event reports, and social media discussions. Their learning capabilities enable rapid detection of safety signals or emerging patient needs, allowing companies to refine product positioning and lifecycle management strategies.
These applications underscore how adaptive AI agents are not just operational tools but strategic assets driving innovation and growth in pharmaceutical enterprises.
AI Agents Are Cost-effective
AI agents are redefining cost efficiency in pharmaceutical commercial operations by enabling smarter resource allocation, automating high-cost processes, and optimizing decision-making across the value chain.
One application lies in dynamic salesforce optimization, where AI agents analyze territory-specific prescribing data, market dynamics, and healthcare provider (HCP) engagement history to recommend optimal deployment of sales representatives. This ensures that high-value physicians receive targeted attention while reducing unnecessary field visits, ultimately cutting operational costs.
Another innovative use case is in adaptive promotional material management, where AI agents assess real-time market feedback and prescription trends to automatically update or retire underperforming marketing assets, minimizing wasteful expenditure on ineffective campaigns.
Additionally, AI agents streamline pricing and reimbursement negotiations by processing payer data and historical pricing trends, enabling pharma companies to identify the most cost-effective pricing strategies that maximize reimbursement potential while maintaining competitive positioning.
In inventory management for commercial launches, AI agents predict demand spikes for new drugs based on patient demographics, competitive activity, and regional healthcare policies, reducing excess production costs or shortages that could lead to lost revenue.
Furthermore, in digital engagement channels, AI agents automate HCP interactions by learning from past engagement patterns and tailoring cost-efficient digital content delivery, significantly lowering the reliance on costly in-person meetings. These agents also optimize multi-channel marketing budgets by dynamically reallocating spend across platforms—such as digital ads, email campaigns, and webinars—based on real-time performance metrics, ensuring maximum ROI from every dollar spent.
AI Agents Revolutionize Collaborative Processes
Moreover, the collaboration between AI and human workers fosters a dynamic where AI handles repetitive tasks or large-scale data analysis, while human experts focus on strategic oversight, nuanced decision-making, and creative problem-solving. This synergy not only boosts productivity but also enhances the strategic thinking within the organization. Teams can explore innovative treatment modalities, engage in predictive analytics for market trends, and tackle complex regulatory landscapes with a blend of human intuition and AI’s computational prowess.
The integration of AI agents into collaborative processes within the pharmaceutical industry marks a significant leap towards operational excellence and innovation. Leveraging APIs and data sources, AI agents facilitate seamless integration and real-time data sharing across various platforms, enabling unprecedented collaboration.
The continuous improvement in collaborative strategies driven by AI not only enhances efficiency but also fosters an environment where strategic thinking and problem-solving are elevated. Teams can now focus on complex, high-value tasks, supported by AI’s data-driven insights, leading to more innovative solutions and better patient outcomes. This synergy between human expertise and AI’s analytical capabilities is setting a new standard for collaborative processes in the pharmaceutical industry, making it more agile, predictive, and ultimately, more effective in meeting the evolving needs of healthcare.
AI Agents Revolutionize Workforce Efficiency
AI agents are radically transforming workforce efficiency in the pharmaceutical industry by enabling more strategic use of human resources. Rather than replacing workers, AI enhances their capabilities, enabling them to focus on high-impact tasks.
In scientific communication and medical information: AI agents can rapidly process complex scientific literature and generate concise summaries for medical science liaisons (MSLs), freeing up their time for higher-value activities like engaging with key opinion leaders (KOLs) and disseminating critical scientific insights.
In market access and payer engagement, AI agents can analyze vast datasets of payer policies, formulary decisions, and clinical trial data to identify optimal negotiation strategies and predict reimbursement outcomes, empowering market access teams to secure favorable coverage decisions and accelerate patient access to new therapies.
Furthermore, in competitive intelligence, AI agents can continuously monitor competitor activities, analyze market trends, and identify emerging threats and opportunities, providing commercial teams with real-time insights that inform strategic planning and competitive positioning.
Even in human resources, AI agents can streamline recruitment processes by screening resumes, conducting initial candidate interviews, and identifying top talent, reducing the workload on HR professionals and accelerating the hiring process. By integrating AI agents into these critical commercial functions, pharmaceutical companies can significantly enhance workforce productivity, optimize resource allocation, and drive greater efficiency across the organization.
The Future of AI Agents
The pharmaceutical industry is on the brink of a new era with the advent of Adaptive Learning Systems within AI agents. These systems go beyond the traditional static models by dynamically evolving in response to new data inputs, clinical trial results, and real-world evidence.
Here’s how this trend will manifest:
Continuous Learning and Adaptation: Unlike previous generations of AI, these adaptive learning systems will not require periodic updates but will instead learn continuously from their environment. This means that as new drugs are developed, new side effects are identified, or new treatment protocols are established, the AI agents will automatically adjust their knowledge base. This capability ensures that the AI remains at the forefront of pharmaceutical knowledge, reducing the lag time between scientific discovery and its application in drug development.
Context-Aware Decision Making: These AI agents will not just process data; they will understand context. For instance, they can differentiate between similar symptoms caused by different diseases or drug interactions, considering patient history, environmental factors, and even socio-economic conditions. This nuanced understanding allows for more precise diagnostic support, treatment recommendations, and personalized medicine strategies, thereby enhancing patient outcomes.
Predictive Model Refinement: With adaptive learning, AI agents will refine their predictive models over time. They will learn from the successes and failures of treatments, optimizing clinical trial designs to focus on the most promising compounds or patient demographics. This iterative learning process reduces the cost and time associated with drug development by focusing resources where they are most likely to yield results.
Proactive Risk Management: Adaptive learning systems will also play a critical role in risk management by predicting potential issues before they arise. By learning from past drug recalls, adverse event reports, and regulatory changes, these AI agents can forecast potential risks associated with new drugs, suggesting modifications in development processes or even alternative compounds to mitigate those risks.
Ethical and Regulatory Alignment: As these systems evolve, they will incorporate ethical decision-making frameworks, aligning with regulatory standards dynamically. This ensures that while innovation is pursued, it is done so within the bounds of ethical practices and regulatory compliance, which is crucial for maintaining public trust and ensuring patient safety.
Conclusion
AI agents are revolutionizing the pharmaceutical industry by automating routine tasks, enhancing collaboration, and providing actionable insights from complex data sets. Their future in pharma is bright, promising personalized medicine, accelerated drug discovery, and optimized operations. Companies must embrace AI to maintain competitiveness, foster innovation, and improve patient outcomes. The time to integrate AI technology is now, for those who do will lead the charge in transforming healthcare delivery and outcomes.
Found this article interesting?
P.S. Here are 5 ways we can help you accelerate your Pharma AI results:
1. Follow Dr Andrée Bates LinkedIn Profile Now
Dr Bates posts regularly about AI in Pharma so if you follow her you will get even more insights.
2. Listen to our AI for Pharma Growth Podcast
Revolutionize your team’s AI solution vendor choice process and unlock unparalleled efficiency and save millions on poor AI vendor choices that are not meeting your needs! Stop wasting precious time sifting through countless vendors and gain instant access to a curated list of top-tier companies, expertly vetted by leading pharma AI experts.
Every year, we rigorously interview thousands of AI companies that tackle pharma challenges head-on. Our comprehensive evaluations cover whether the solution delivers what is needed, their client results, their AI sophistication, cost-benefit ratio, demos, and more. We provide an exclusive, dynamic database, updated weekly, brimming with the best AI vendors for every business unit and challenge. Plus, our cutting-edge AI technology makes searching it by business unit, challenge, vendors or demo videos and information a breeze.
Discover vendors delivering out-of-the-box AI solutions tailored to your needs.
Identify the best of the best effortlessly.
Anticipate results with confidence.
Transform your AI strategy with our expertly curated vendors that walk the talk, and stay ahead in the fast-paced world of pharma AI!
Get on the wait list to access this today. Click here.
4. Take our FREE AI for Pharma Assessment
When we analysed the most successful AI in biopharma and their agencies, we found there are very specific strategies that deliver the most consistent results year after year. This assessment is designed to give clarity as to how to achieve a successful outcome from AI.
The first step is to complete this short questionnaire, it will give us the information to assess which process is right for you as a next step.
It’s free and obligation-free, so go ahead and complete it now. Plus receive a free link to our a free AI tools pdf and our 5 day training (30 mins a day) in AI in pharma. Link to assessment here.
5. Learn more about AI in Pharma in your own time
We have created an in-depth on-demand training about AI specifically for pharma that translate it into easy understanding of AI and how to apply it in all the different pharma business units — Click here to find out more.