In the crucible of modern drug development—where a single molecule’s journey from lab to patient spans an average of 12 years and $2.3 billion—AI has emerged as pharma’s most potent ally, compressing discovery timelines, predicting clinical trial failures, and unlocking precision therapies for once-untreatable diseases.
Yet, as C-suites race to harness algorithms that could redefine competitive advantage, a stark disconnect persists: over 60% of pharma teams confess to sidelining AI tools and clinging to spreadsheets and legacy workflows despite glaring inefficiencies. This paradox isn’t rooted in technology’s limitations but in a more profound, often unspoken tension—the collision of innovation with institutional inertia.
Having spearheaded AI integrations across pharma giants, I’ve witnessed how resistance festers not from scepticism of AI’s potential but from misaligned incentives, regulatory ambiguity and a workforce fearing obsolescence.
Here, we dissect this friction and deliver a blueprint to transform resistors into champions, aligning AI’s computational brilliance with the irreplaceable human ingenuity that fuels this industry’s noblest missions.
Why Do Employees Resist AI? Unpacking the Root Causes
A) Fear of Job Displacement
Within pharmaceutical environments, the fear of job displacement runs deeper than mere technological anxiety—it represents an existential threat to highly specialized career paths built over decades. Research scientists who have dedicated 15-20 years to developing domain expertise in specific therapeutic areas perceive AI as devaluing their hard-earned intuition and experimental design capabilities. This fear manifests differently across organizational hierarchies: medicinal chemists worry about AI-driven molecule generation replacing iterative human design; clinical trial managers fear automated protocol development; regulatory specialists see their judgment being supplanted by algorithmic compliance checks.
The resistance is particularly pronounced at mid-career levels where professionals have invested heavily in specialized knowledge but lack the positional security of senior leadership. Unlike other industries, pharmaceutical displacement anxiety is compounded by the limited transferability of highly specialized skills—an oncology researcher focused on kinase inhibitors cannot easily pivot to consumer technology if displaced. This creates a self-reinforcing cycle where those most threatened become the most vocal opponents during implementation phases, often subtly undermining adoption through passive non-compliance rather than direct opposition.
Most organizational change management frameworks fail to recognize the unique psychological contract between pharmaceutical professionals and their specialized domains—where identity is deeply intertwined with scientific expertise. When AI systems begin generating insights previously requiring years of human judgment, the resulting cognitive dissonance manifests as resistance framed as “quality concerns” rather than acknowledged job insecurity.
B) Lack of Understanding
The pharmaceutical knowledge gap around AI extends beyond general technical illiteracy—it reflects a fundamental disconnect between two disparate epistemological traditions. Pharmaceutical research has historically operated through hypothesis-driven experimentation, mechanistic understanding, and causal relationships, while machine learning thrives on pattern recognition and statistical correlations without necessarily revealing underlying mechanisms. This philosophical tension creates profound discomfort among scientists trained to value explanatory models over predictive ones.
Cross-disciplinary communication failures exacerbate this divide. Data scientists deploy terminology (neural networks, backpropagation, dimensionality reduction) that sounds abstract to biologically-trained researchers, while pharmaceutical experts speak in equally opaque jargon (pharmacokinetics, allosteric modulation, adaptive immunity) incomprehensible to computational teams. The resulting mutual incomprehension breeds mistrust, as neither group can effectively validate the other’s expertise.
This understanding deficit is compounded by asymmetric risk perceptions. IT and data science teams view failed AI implementations as iterative learning opportunities, while pharmaceutical researchers perceive the same failures through the lens of patient safety and regulatory risk. Without a shared framework for evaluating outcomes, both sides speak past each other, reinforcing the perception that AI proponents “don’t understand” the true complexities of pharmaceutical development.
C) Trust and Transparency Concerns
In pharmaceutical environments, AI transparency issues transcend general algorithmic “black box” concerns—they directly challenge the industry’s foundational regulatory paradigm of demonstrable causality and documented decision chains. Scientific and regulatory professionals trained in Good Laboratory, Manufacturing, and Clinical Practices (GxP) environments operate within validation frameworks that demand exhaustive documentation of methodologies, clear audit trails, and reproducible decision processes. When confronted with deep learning systems whose internal representations remain indecipherable even to their creators, these professionals experience profound cognitive discomfort that manifests as resistance.
Regulatory compliance concerns compound this resistance, particularly in validation-critical functions like manufacturing and quality control. Decision-makers accountable to health authorities rightfully question how they will defend AI-derived conclusions during regulatory inspections when they cannot fully articulate the system’s reasoning. This creates a paradoxical situation where the most sophisticated AI applications face the strongest resistance in the very domains where they could provide the greatest value.
The trust deficit extends to data provenance and representational concerns. Pharmaceutical professionals intimately familiar with the limitations of real-world healthcare data—fragmentation, documentation gaps, coding inconsistencies, and sampling biases—correctly question whether AI systems adequately account for these limitations or simply propagate and amplify existing biases. Without transparent mechanisms to evaluate these concerns, resistance becomes the default protection mechanism against perceived regulatory and scientific risks.
D) Past Technological Failures
The pharmaceutical industry carries a collective organizational memory of spectacular technological disappointments that colours all subsequent innovation reception. Early electronic data capture systems promised to revolutionize clinical trials but initially delivered clunky interfaces that doubled documentation workloads. Early computational chemistry platforms predicted candidate molecules that systematically failed in actual biological systems. First-generation laboratory automation frequently broke down mid-experiment, destroying irreplaceable samples and delaying critical programs.
These historical failures resonate differently within pharmaceutical organizations than in other sectors because of the high-stakes, long-timeline nature of drug development. A failed customer relationship management system implementation in retail might cost money and time; a failed laboratory informatics platform in pharma can derail a decade-long development program representing hundreds of millions in investment. This asymmetric risk profile creates institutional trauma that persists across leadership changes and organizational restructuring.
Significantly, pharmaceutical professionals have witnessed waves of overhyped technologies that promised paradigm shifts but delivered incremental improvements at best—combinatorial chemistry, high-throughput screening, systems biology, and rational drug design all arrived with revolutionary rhetoric but resulted in evolutionary progress. This experience has conditioned a reflexive scepticism toward transformational technology claims—scepticism that AI advocates must acknowledge and address rather than dismiss as resistance to change.
E) Organizational Inertia
Pharmaceutical organizational inertia manifests through unique structural characteristics that actively resist technological transformation. The industry’s bifurcated leadership model—with parallel scientific and business hierarchies—creates competing decision frameworks that must both align for successful AI adoption. Scientific leadership evaluates AI through evidential standards designed for therapeutic interventions, demanding levels of validation inappropriate for operational technologies. Meanwhile, business leadership applies financial metrics ill-suited to capturing AI’s long-term transformational potential.
This inertia is institutionalized through validation and documentation requirements that originated for patient safety but expanded to encompass all systems touching regulated processes. Simple algorithm updates that would require days in technology companies can require months in pharmaceutical environments due to change control procedures, documentation requirements, and validation protocols. These legitimate quality processes become powerful inertial forces when applied uncritically to AI systems designed for continuous learning and improvement.
Cross-functional dependencies further complicate adoption. A seemingly straightforward AI application in pharmacovigilance might require alignment across clinical operations, regulatory affairs, safety, legal, IT, and therapeutic area teams—each with different priorities, success metrics, and risk tolerances. The resulting coordination tax means pharmaceutical AI initiatives face substantially higher organizational friction than identical technologies in less regulated industries, creating the appearance of resistance when the actual challenge is complex cross-functional orchestration.
F) Ethical and Cultural Apprehensions
The pharmaceutical industry’s ethical resistance to AI stems from deeply encoded cultural values around patient centricity and scientific integrity that manifest in organization-specific ways. Research-driven organizations prize scientific causality and mechanistic understanding, viewing AI’s pattern-recognition approach as epistemologically suspect despite its predictive power. Patient-centred organizations worry that algorithmic decision support will erode the human judgment essential to compassionate care. Quality-focused organizations see AI’s probabilistic outputs as fundamentally incompatible with zero-defect manufacturing standards.
These concerns reflect legitimate tensions between pharmaceutical and technology value systems. The “move fast and break things” ethos that drives technological innovation directly conflicts with the “proceed with caution and verify everything” mindset essential to safe drug development. When AI advocates frame resistance as simple technophobia, they miss this deeper value conflict—pharmaceutical professionals are not resisting change but defending core principles they correctly recognize as essential to their societal mission.
Leadership’s ethical framing significantly impacts adoption trajectories. Organizations that position AI as augmenting human capabilities rather than replacing human judgment see dramatically lower resistance. However, this framing must be authentic—pharmaceutical professionals quickly detect disconnects between leadership rhetoric about “human-centred AI” and implementation realities that devalue human expertise. This authenticity gap explains why seemingly identical AI initiatives thrive in some pharmaceutical organizations while failing in others despite similar technical approaches.
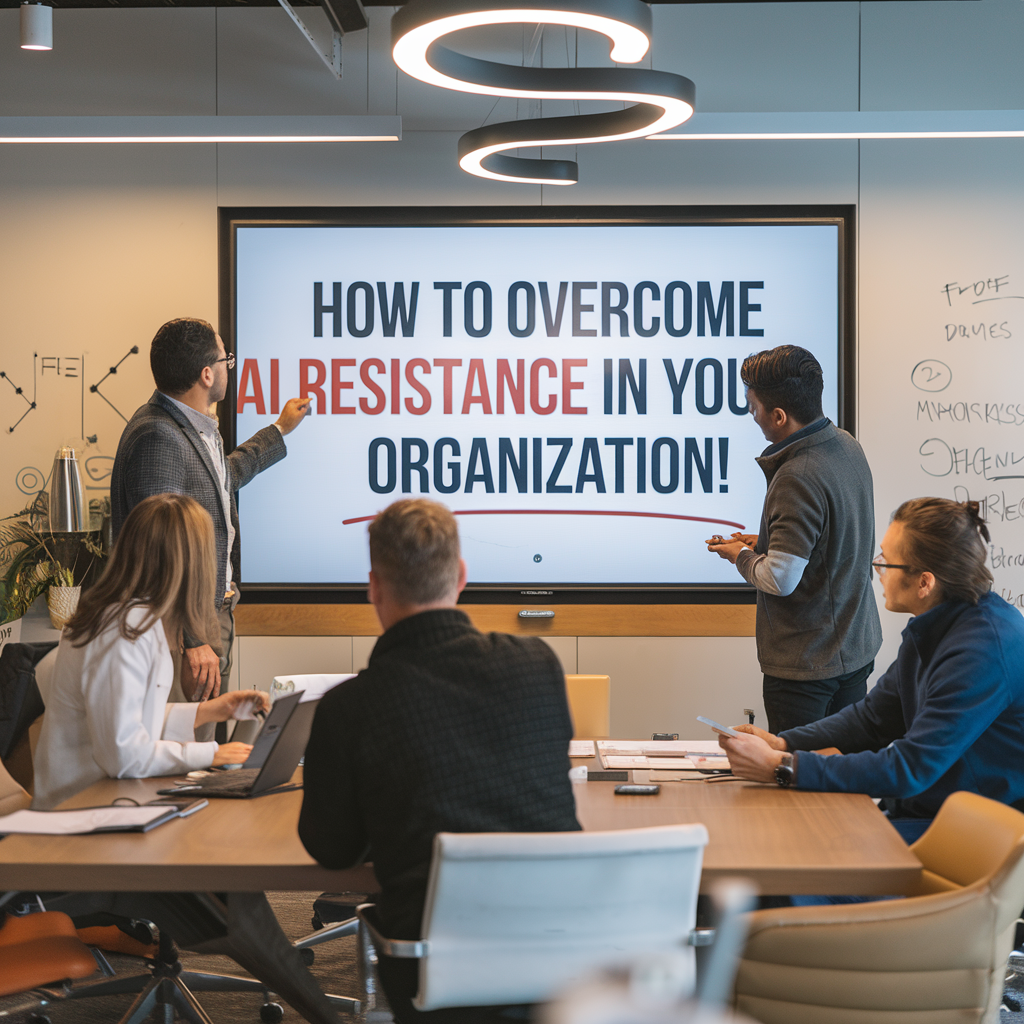
Addressing Resistance: Strategies to Bring Teams Along
1) Combat Fear with Upskilling and Role Evolution
Pharmaceutical organizations must reframe AI adoption as an evolution of expertise rather than a displacement event. This begins by identifying high-value tasks where AI amplifies human judgment—for instance, enabling clinical researchers to shift from manual adverse event reporting to interpreting AI-curated safety signals for strategic trial adjustments.
Reskilling programs should be role-specific and tiered: bench scientists need training in AI-assisted target identification, while medical writers require NLP tool mastery for automated literature synthesis. Crucially, these programs must validate existing expertise—positioning AI fluency as an enhancement to, not a replacement for, domain mastery.
New roles like “AI translators” (bridging computational and scientific teams) or “algorithm stewards” (monitoring model drift in validated systems) create career pathways that align with pharma’s regulated environment. By publicly promoting early adopters into these hybrid roles, organizations signal that AI proficiency is a leadership competency, not a threat.
2) Demystify AI Through Education
Education initiatives must dissolve the abstraction layer separating scientists from AI’s mechanics. Interactive workshops should use therapeutic-area-specific datasets—for example, having oncology teams build predictive models for drug response using their own trial data. This hands-on approach reveals AI as an extension of the scientific method rather than a foreign entity.
Success stories should emphasize process over outcomes: detailing how a pharmacokinetics team reduced variability in dose optimization by collaborating with ML engineers, rather than touting generic efficiency gains. Internal “AI journals” documenting failed experiments and iterative improvements can normalize trial-and-error learning, mirroring the R&D culture that pharmaceutical professionals already respect.
3) Build Trust via Transparency
In pharma, trust is built through audit trails, not algorithms. Every AI system must produce decision logs that mirror the documentation rigor of clinical study reports. For instance, a predictive model forecasting trial enrolment should output not just predictions but confidence intervals, feature weightings, and sensitivity analyses—formats familiar to regulatory reviewers.
Cross-functional co-creation is non-negotiable: when developing an AI tool for medical affairs, involve KOLs in training data curation to ensure the model reflects real-world clinical nuance. Implement “glass box” review boards where stakeholders validate AI outputs against manual analyses, creating empirical evidence of reliability. This transparency extends to data lineage—provenance tracking that satisfies pharmacovigilance requirements while demonstrating ethical data stewardship.
4) Start Small, Scale Gradually
Initial pilots must align with the industry’s risk-averse ethos. Many areas can deliver quick wins – such as automating pharmacovigilance case processing (a high-volume, rules-driven task) – without threatening core scientific roles. Subsequent phases should target “adjacent innovations”—like using AI to optimize comparator drug sourcing in trials, a pain point familiar to all but not mission-critical.
Each success is a teachable moment: a pilot reducing CMC documentation errors by X% becomes a workshop blueprint, dissecting how AI caught human-overlooked inconsistencies.
Scale-up follows a validation cadence pharma trust: after proving reliability in GLP environments, deploy AI in GCP settings with pre-defined human override protocols.
5) Foster Leadership Alignment
Pharma’s dual leadership structure (scientific and commercial) demands tailored engagement. For CSOs, position AI as a force multiplier in the race for first-in-class therapies—showcasing how AlphaFold-like tools accelerate target validation. CFOs respond to AI’s potential to shave months from development timelines, directly impacting NPV.
Establish an AI governance council co-chaired by R&D and compliance leaders, mandating monthly reviews of use cases against predefined KPIs (model accuracy, protocol deviations, audit readiness). Break silos through rotational programs: IT engineers embed in clinical teams to co-develop monitoring algorithms, while statisticians rotate into AI labs to stress-test model assumptions.
6) Address Ethical Concerns Proactively
Pharma’s AI ethics frameworks must exceed general corporate standards, embedding patient safety into every layer. This means bias mitigation protocols that account for underrepresented populations in training data—not just racial diversity but rare disease cohorts and elderly polypharmacy patients.
Establish ethics review checkpoints mirroring IRB processes: before deploying an AI tool in trials, an interdisciplinary panel (clinicians, ethicists, patient advocates) evaluates its impact on informed consent, data privacy, and equitable access. Publish internal “algorithm impact assessments” detailing how models handle edge cases like off-label usage signals or paediatric data extrapolation. By aligning AI governance with existing pharmaco-ethical frameworks (e.g., ICH GCP principles), organizations transform compliance from a barrier into a trust-building asset.
Sustaining Excitement and Learning
A) Gamify AI Adoption
Pharmaceutical organizations must engineer AI engagement through purpose-built challenges that mirror real-world scientific and commercial dilemmas. Instead of generic hackathons, structure competitions around therapeutic-area-specific problems—challenging teams to predict compound toxicity using historical data or optimize patient recruitment for rare disease trials.
Reward systems should mirror pharma’s achievement culture: winning solutions gain fast-tracked pilot funding, publication in internal research repositories, or presentation opportunities at global R&D summits.
Badge ecosystems must align with professional identities—a “GenAI Compliance Master” certification for regulatory affairs carries more weight than generic AI literacy badges. Crucially, gamification succeeds when tied to career capital: participation becomes a visible marker of leadership potential in innovation-driven promotion cycles.
B) Create AI Champions
The most effective AI champions in pharma are not technologists but respected domain experts who’ve successfully integrated AI into their workflows. Identify early adopters like senior clinical pharmacologists using ML to model drug-drug interactions or medical affairs leaders leveraging NLP for KOL sentiment analysis.
Empower these champions through “AI Ambassador” roles with dedicated time to mentor peers, emphasizing their dual credibility in science and technology. Internal storytelling must highlight the process of adoption—how a veteran toxicologist overcame scepticism by validating AI predictions against 20 years of histopathology reports.
Scale influence through cross-functional “AI Grand Rounds,” where champions present use cases in the same forums used for clinical trial updates, signalling equal strategic importance.
C) Continuous Learning Pathways
Continuous learning in pharma must transcend generic upskilling platforms. Curate AI curricula that intersect with therapeutic priorities—oncology teams access deep dives on AI in biomarker discovery, while commercial teams’ study predictive analytics for launch excellence.
Partner with academic medical centres to co-develop micro-credentials that blend AI fundamentals with Good Machine Learning Practice (GMLP) standards. Lunch-and-learns gain traction when featuring translational experts—regulatory consultants who’ve navigated AI-based submission approvals or computational biologists bridging academic research and industrial applications.
Crucially, learning pathways must offer tiered progression: a medical writer starts with AI-assisted literature synthesis, advances to protocol automation, then mentors others—a visible journey reinforcing lifelong learning as career currency.
D) Link AI to Purpose
Pharma’s mission-driven culture demands explicit connections between AI and patient impact. Frame AI tools as “digital co-pilots” accelerating the path from bench to bedside—a molecule generated by AI isn’t just efficient, it’s a potential lifeline for patients awaiting novel therapies.
Internal campaigns should humanize data: “Every hour saved by AI-driven adverse event analysis = 10 additional patients enrolled in our lupus trial.” For commercial teams, reposition AI as a force multiplier for HCP education—algorithmically personalized content ensures time-constrained oncologists receive the precise data needed to inform treatment decisions. This purpose alignment must permeate metrics: Include patient advocacy groups in AI impact assessments and feature patient stories in AI update forums.
E) Measure and Communicate Impact
Pharma-specific AI KPIs must speak to both scientific and commercial stakeholders. R&D teams track AI’s impact on target validation cycle times or first-pass IND success rates. Manufacturing monitors AI-driven yield improvements against ICH Q10 benchmarks.
Commercial units measure AI-optimized campaign engagement against traditional physician outreach. Centralized dashboards should adopt the visual language of clinical trial reports—forest plots comparing AI-assisted vs. manual outcomes, survival curves depicting error reduction over time. In town halls, contextualize metrics within familiar frameworks: “Our AI-assisted literature review matches the rigor of a systematic Cochrane review, but completes it ‘Y’x faster, accelerating protocol development.”
F) Future-Proof the Workforce
Agility in pharma hinges on embedding AI fluency into professional identity. Reframe learning as a GxP-like competency—just as GCP training is mandatory for clinical roles, AI literacy becomes essential for protocol designers or medical science liaisons.
Rotational programs must facilitate bidirectional knowledge flow: data scientists embed in clinical operations to grasp endpoint adjudication complexities, while medical directors rotate into AI labs to stress-test models against real-world patient heterogeneity. Career architectures should reward hybrid expertise—promotion criteria for therapeutic area heads include mentoring AI initiatives, while lab directors are evaluated on computational collaboration. This creates an ecosystem where AI isn’t a disruption but an intrinsic element of pharma’s evolving expertise landscape.
Conclusion
Resistance to AI in pharma—rooted in fear of displacement, opaque algorithms, and institutional inertia—is surmountable through strategies that honour the industry’s scientific rigor and ethical imperatives.
Begin with targeted pilots in areas like clinical trial optimization or pharmacovigilance to demonstrate AI’s role as a collaborator, not a disruptor. Invest in hybrid talent development, blending domain expertise with AI literacy while anchoring initiatives to organizational values like patient safety and innovation. Crucially, AI adoption should be viewed not as a checkbox exercise but as a cultural metamorphosis: one that prioritizes curiosity to explore computational frontiers and resilience to navigate inevitable setbacks. The true measure of success lies not in algorithms deployed but in teams empowered to reimagine therapeutic discovery through human-machine symbiosis.