The pharmaceutical industry is at the forefront of innovation, constantly pushing the boundaries to improve patient care and advance scientific breakthroughs. In this era of progress, Artificial Intelligence (AI) has emerged as a game-changer, offering unprecedented opportunities to revolutionize pharmaceutical research, development, and patient outcomes. From expediting drug discovery processes to optimizing personalized medicine, AI presents a vast array of possibilities.
However, initiating AI projects within the pharma sector entails navigating a unique set of challenges and risks. Key considerations such as regulatory compliance, data security, and aligning AI approaches with specific business needs demand meticulous planning and flawless execution.
In this comprehensive article, we delve into the intricacies of planning AI projects in the pharmaceutical industry, focusing on the vital roles of AI deployment blueprint and project scoping.
Business, Functional, and Usability Requirements
Importance of aligning the proposed approach with business user requirements
When planning an AI project blueprint in the pharmaceutical industry, aligning AI projects with the specific requirements of businesses is of paramount importance. By tailoring AI solutions to address the unique needs and objectives of pharmaceutical companies, organizations can unlock significant value and gain a competitive advantage.
One notable example of aligning AI deployment blueprints with business user requirements is the use of predictive analytics in drug discovery. Pharmaceutical companies generate vast amounts of data during the drug development process, including molecular data, clinical trial data, and real-world evidence. By leveraging AI algorithms, these companies can analyze this data to identify patterns, predict drug efficacy, and optimize the selection of candidate compounds. This approach significantly accelerates the drug discovery process, saving time and resources, and ultimately brings life-saving medications to patients faster.
According to a report by PwC, the adoption of AI technologies in the healthcare industry, including pharmaceuticals, has the potential to create $150 billion in annual savings by 2026. This demonstrates the transformative power of aligning AI projects with business user requirements, as it can drive operational efficiency, cost reduction, and improved patient outcomes.
Identifying and addressing functional and usability requirements
In addition to aligning with business user requirements, identifying and addressing functional and usability requirements are crucial steps in planning successful AI projects in the pharma industry.
Functional requirements refer to the specific capabilities and features that the AI system should possess to meet the intended goals. AI deployment blueprints can provide guidance in defining these functional requirements.
For example, in clinical decision support systems, the AI solution should have the ability to process patient data, recommend treatment options, and provide accurate predictions. By understanding the functional requirements outlined in the AI blueprint, pharmaceutical companies can select or develop AI algorithms and models that effectively fulfill these needs.
Usability requirements focus on ensuring that the AI solution is intuitive, user-friendly, and seamlessly integrates into existing workflows. This involves considering factors such as the user interface, ease of data input, interpretability of results, and the ability to customize the system based on user preferences. A well-designed and user-centric AI solution, as outlined in the AI deployment blueprint, can increase user adoption, improve efficiency, and enhance overall user satisfaction.
An example of addressing usability requirements is the development of AI-powered electronic health record (EHR) systems. These systems aim to simplify data entry and retrieval, automate documentation, and provide real-time clinical decision support to healthcare professionals. By designing EHR systems with a user-centric approach, healthcare providers can reduce administrative burdens, improve patient care quality, and enhance overall productivity.
To ensure that functional and usability requirements are met, organizations can conduct user research, usability testing, and iterative design processes. These practices help gather feedback from stakeholders and refine the AI solution iteratively, resulting in a more effective and user-friendly implementation. The AI deployment blueprint serves as a valuable reference throughout these processes, guiding the development and deployment of the AI solution.
Data Identification and Planning
A) Data access and API investigation for effective data integration
Data access and integration play a critical role in AI projects within the pharma industry. It involves identifying relevant data sources and exploring the availability of application programming interfaces (APIs) for seamless data integration into the AI system as outlined in the AI deployment blueprint.
For example, pharmaceutical companies may need access to diverse datasets, such as electronic health records, clinical trial data, genomics data, and scientific literature. Through APIs, as specified in the AI blueprint they can establish connections with various data repositories and leverage the power of AI to extract valuable insights.
One noteworthy example is the collaboration between pharma giant Pfizer and Flatiron Health, a healthcare technology company. Pfizer gained access to de-identified clinical data from Flatiron’s oncology electronic health records database through an API, as detailed in the AI deployment blueprint and AI blueprint. This integration enabled Pfizer to conduct real-world evidence studies and gain insights into patient outcomes, treatment responses, and disease progression. The use of APIs facilitated the seamless integration of data into Pfizer’s AI systems, as described in the AI blueprint, enhancing their ability to generate meaningful insights.
B) Ensuring data regulatory and legal compliance
Data regulatory and legal compliance is crucial in the pharma industry, where privacy and confidentiality of patient information are paramount. When implementing AI projects, pharmaceutical companies must adhere to regulations such as the Health Insurance Portability and Accountability Act (HIPAA) and the General Data Protection Regulation (GDPR), as outlined in the AI blueprint.
For instance, when handling patient data, pharmaceutical companies must ensure proper anonymization, secure storage, and restricted access, as specified in the AI deployment blueprint and AI blueprint. They need to establish robust data protection measures to safeguard sensitive information throughout the data lifecycle. Compliance with these regulations, as outlined in the AI deployment blueprint, helps build trust with patients, healthcare providers, and regulatory authorities.
C) Identifying gaps in data availability and quality
In many cases, there may be gaps in the availability and quality of data required for AI projects in the pharma industry. Identifying these gaps is essential to understand the limitations and potential biases in the data used for training AI models.
For instance, if a pharmaceutical company aims to develop an AI algorithm for personalized medicine, it requires a diverse dataset that represents different demographics, genetic variations, and disease conditions. Identifying gaps in data availability allows organizations to strategize ways to collect missing data or explore alternative approaches, such as data augmentation techniques or partnerships with other healthcare institutions.
D) Establishing an optimal data approach for the project
Establishing an optimal data approach involves designing a framework for data collection, storage, preprocessing, and management. It includes determining the data formats, structuring data pipelines, and establishing protocols for data cleaning and validation.
For instance, a pharmaceutical company planning to implement AI for adverse event detection may establish data collection processes from various sources such as patient reports, social media, and pharmacovigilance databases, following the guidelines in the AI deployment blueprint and AI blueprint. They would design a data workflow that ensures data quality, consistency, and scalability, as detailed in the AI deployment blueprint.
According to a report by IQVIA, pharmaceutical companies are increasingly recognizing the value of AI and real-world data. The report indicates that the use of AI and real-world evidence can potentially reduce drug development costs by up to 20% and shorten the development timeline by up to 18 months.
By conducting thorough investigations of data access, ensuring compliance with regulations as outlined in the AI deployment blueprint and AI blueprint, addressing data gaps, and establishing an optimal data approach, pharmaceutical companies can lay a solid foundation for successful AI projects. This enables them to leverage the power of data-driven insights to accelerate drug discovery, improve clinical decision-making, and ultimately enhance patient care, as specified in the AI blueprint.
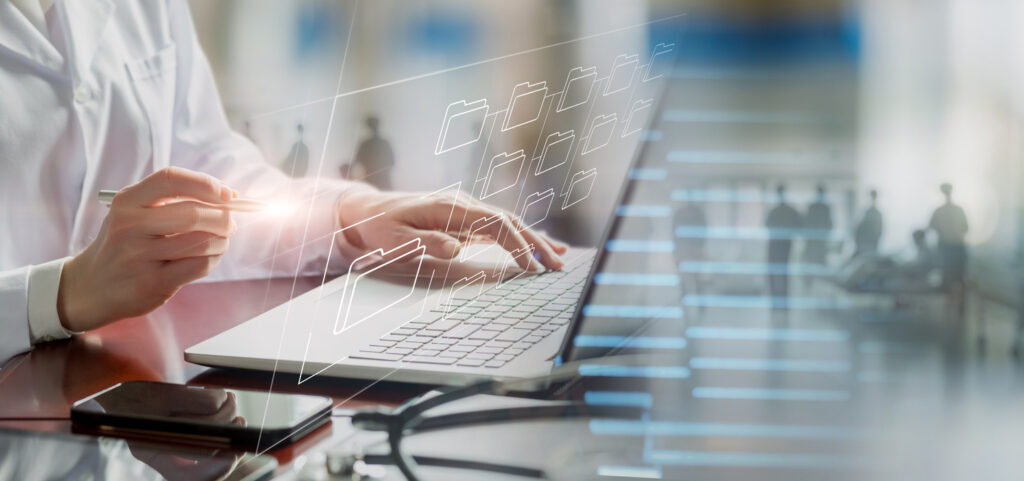
Risk and Dependency Identification
A. Understanding the potential risks associated with AI projects
AI projects in the pharma industry, like any other complex initiative, come with inherent risks. It is crucial to have a comprehensive understanding of these risks to ensure project success.
Some potential risks associated with AI projects in pharma include:
1. Data quality and integrity: Poor data quality, incompleteness, or biases in the training data can adversely impact the accuracy and reliability of AI models.
2. Ethical considerations: AI algorithms must comply with ethical guidelines, ensuring fairness, transparency, and accountability in decision-making processes. Failure to address ethical concerns can lead to reputational damage and legal repercussions.
3. Regulatory compliance: Compliance with regulatory frameworks, such as HIPAA and GDPR, is essential to protect patient privacy and maintain legal compliance when handling sensitive healthcare data.
4. Technical limitations: AI models may have limitations, such as interpretability challenges, difficulty in handling complex data types, or limitations in scalability, which can impact their effectiveness and usability.
B. Strategies for identifying and mitigating project risks
To ensure the success of AI projects in the pharma industry, it is essential to identify and mitigate risks.
Here are some strategies for risk management:
1. Conduct a thorough risk assessment: Identify and analyze potential risks specific to the project, considering technical, regulatory, operational, and security aspects.
2. Implement robust data governance: Establish data quality control measures, address bias, ensure data privacy, and maintain compliance with applicable regulations.
3. Rigorous model validation and testing: Perform comprehensive validation and testing of AI models to ensure accuracy, reliability, and generalizability.
4. Engage domain experts and stakeholders: Involve experts from various domains, including healthcare professionals, regulatory experts, and legal advisors, to assess risks and provide insights.
5. Develop a contingency plan: Anticipate potential risks and develop contingency plans to mitigate their impact, ensuring business continuity and minimizing disruptions.
C. Addressing dependencies and creating contingency plans
AI projects often have dependencies on various factors, including data availability, technological infrastructure, external collaborations, and regulatory approvals. It is crucial to identify these dependencies and develop contingency plans to mitigate any potential issues.
For example, if an AI project relies on external data sources, such as collaborations with research institutions or access to third-party datasets, it is important to establish clear communication channels, legal agreements, and backup options in case of unavailability or delays, as outlined in the AI blueprint.
Furthermore, regulatory approvals and compliance can significantly impact project timelines. By proactively engaging with regulatory bodies and understanding the requirements, as specified in the AI deployment blueprint, pharmaceutical companies can plan for potential delays and ensure adherence to regulations.
Addressing dependencies and creating contingency plans helps mitigate the impact of unforeseen events, ensuring project progress and minimizing disruptions, as detailed in the AI deployment blueprint and AI blueprint.
By understanding potential risks, implementing risk mitigation strategies, as outlined in the AI deployment blueprint, and addressing project dependencies, pharmaceutical companies can increase the likelihood of successful AI project implementation. Proactive risk management, as specified in the AI blueprint, fosters a secure and reliable environment for leveraging the transformative power of AI in the pharma industry.
Legal and Compliance Approach
A) Importance of complying with laws and regulations in the pharma industry
Compliance with laws and regulations is of utmost importance in the pharmaceutical industry. The nature of AI projects in healthcare, including drug discovery, clinical trials, and patient care, necessitates strict adherence to legal and ethical guidelines. Failure to comply can lead to severe consequences, including legal penalties, reputational damage, and loss of public trust.
1. Intellectual property rights: Pharmaceutical companies must respect and protect intellectual property rights when utilizing AI technologies. This includes ensuring proper licensing, avoiding copyright infringement, and respecting patent rights.
2. Privacy and data protection: The use of patient data in AI projects requires compliance with regulations such as HIPAA (in the United States) and GDPR (in the European Union). These regulations mandate the secure handling, storage, and transfer of patient information to protect privacy and ensure data confidentiality.
3. Ethical considerations: AI projects in the pharma industry must address ethical concerns, such as informed consent, transparency, and fairness. Ensuring that AI algorithms do not perpetuate biases and are used responsibly is essential to maintain ethical standards.
B) Ensuring the project adheres to legal and ethical guidelines
To ensure legal and ethical compliance in AI projects, pharmaceutical companies should undertake several measures:
1. Conduct thorough legal assessments: Evaluate the legal landscape and regulatory requirements relevant to the project. Engage legal experts to ensure compliance with intellectual property laws, privacy regulations, and ethical guidelines.
2. Establish data governance frameworks: Implement robust data governance practices, including data anonymization, access controls, and audit trails, to protect patient privacy and comply with data protection regulations.
3. Develop and enforce ethical guidelines: Establish ethical guidelines and principles for AI projects, including transparency, fairness, and accountability. Ensure that AI algorithms are developed and deployed in a manner that mitigates biases and promotes responsible use.
4. Regularly monitor and update compliance: Stay abreast of evolving legal and regulatory changes in the pharma industry. Conduct periodic audits to assess compliance and make necessary updates to policies and procedures.
It is crucial to note that regulatory requirements may vary across countries and regions. Pharmaceutical companies must navigate these complexities and tailor their legal and compliance approach accordingly.
Technical High-Level Workflow
A. Identifying the essential components of the tech stack
Building an effective AI project in the pharma industry requires careful consideration of the technical components that make up the tech stack. The tech stack comprises the software, tools, and infrastructure needed to support data processing, analysis, and visualization.
Some essential components of the tech stack in AI projects include:
1. Data storage: Determine the optimal data storage solution that can handle the volume, velocity, and variety of data generated in the pharma industry. This may involve utilizing cloud-based storage systems, distributed file systems, or databases tailored for big data analytics.
2. Data management: Implement data management systems that enable efficient organization, retrieval, and manipulation of data. This includes data indexing, metadata management, and version control to ensure data integrity and traceability.
3. Data ingestion: Develop mechanisms for seamlessly ingesting data from various sources into the AI system. This may involve data integration frameworks, data pipelines, and ETL (Extract, Transform, Load) processes to cleanse and transform the data for analysis.
4. Data processing: Utilize technologies for processing large-scale datasets, such as distributed computing frameworks (e.g., Apache Spark) or specialized hardware accelerators (e.g., GPUs). These technologies enable efficient data processing, feature extraction, and model training.
5. Data visualization and outputs: Implement tools and libraries for visualizing and interpreting the results of AI models. This may involve interactive dashboards, data visualization libraries (e.g., Plotly, Matplotlib), and reporting frameworks to communicate insights effectively.
6. Client end tools: Consider the user interface and tools that will be used by stakeholders to interact with the AI system. This may include developing user-friendly web applications, mobile apps, or APIs to facilitate seamless user experience and access to AI-driven insights.
B) Exploring data storage, management, ingestion, processing, visualization, and client-end tools
Each component of the technical stack plays a vital role in the success of AI projects in the pharma industry.
Here are some considerations for each component:
1. Data storage: Evaluate options such as cloud-based storage solutions (e.g., Amazon S3, Google Cloud Storage) or on-premises database systems (e.g., PostgreSQL, MongoDB) based on factors like scalability, security, and cost-effectiveness.
2. Data management: Explore data management tools like Apache Airflow or Kubernetes to orchestrate data pipelines, ensure data quality, and automate data workflows.
3. Data ingestion: Assess the availability and compatibility of APIs or connectors to acquire data from various sources, such as electronic health records, clinical trial databases, or wearable devices.
4. Data processing: Consider frameworks like TensorFlow or PyTorch for machine learning model development and training. Distributed computing platforms like Apache Spark may be useful for handling big data processing tasks.
5. Data visualization and outputs: Explore visualization libraries like Matplotlib, Tableau, or Power BI for creating interactive and insightful visual representations of data and analysis results.
6. Client end tools: Depending on the project’s requirements, consider developing web-based interfaces, mobile applications, or integrating AI functionalities into existing software platforms commonly used in the pharma industry.
C) Evaluating the suitability and compatibility of different technologies
When planning the technical high-level workflow, it is crucial to evaluate the suitability and compatibility of different technologies.
Consider factors such as
1. Scalability: Ensure that the chosen technologies can handle large volumes of data and scale as the project expands. This is particularly important in the pharma industry, where datasets can be vast and continuously growing.
2. Integration capabilities: Assess the compatibility of the selected technologies with existing systems, databases, and infrastructure within the organization. Seamless integration minimizes disruptions and enhances workflow efficiency.
3. Performance: AI projects require high-performance technologies to process and analyze data in a timely manner. For example, GPUs (Graphics Processing Units) are widely used in AI applications due to their parallel processing capabilities, enabling faster model training and inference.
4. Compatibility with existing infrastructure: Evaluate the compatibility of the selected technologies with the existing IT infrastructure within the pharma organization. Integration challenges can arise if the chosen technologies are not compatible with the current systems and workflows, potentially leading to inefficiencies and data silos.
5. Community support and documentation: Assess the level of community support, availability of documentation, and resources for the chosen technologies. Robust community support ensures access to forums, tutorials, and updates, which can significantly aid in troubleshooting and optimizing the implementation.
For example, if real-time data processing is crucial, Apache Kafka’s streaming capabilities may be a suitable choice. If data visualization needs to be highly interactive and customizable, JavaScript libraries like D3.js can provide rich visualizations.
This article is part 1 of a series of two parts.
Found this article interesting?
P.S. Here are 5 ways we can help you accelerate your Pharma AI results:
1. Follow Dr Andrée Bates LinkedIn Profile Now
Revolutionize your team’s AI solution vendor choice process and unlock unparalleled efficiency and save millions on poor AI vendor choices that are not meeting your needs! Stop wasting precious time sifting through countless vendors and gain instant access to a curated list of top-tier companies, expertly vetted by leading pharma AI experts.
Every year, we rigorously interview thousands of AI companies that tackle pharma challenges head-on. Our comprehensive evaluations cover whether the solution delivers what is needed, their client results, their AI sophistication, cost-benefit ratio, demos, and more. We provide an exclusive, dynamic database, updated weekly, brimming with the best AI vendors for every business unit and challenge. Plus, our cutting-edge AI technology makes searching it by business unit, challenge, vendors or demo videos and information a breeze.
- Discover vendors delivering out-of-the-box AI solutions tailored to your needs.
- Identify the best of the best effortlessly.
- Anticipate results with confidence.
Transform your AI strategy with our expertly curated vendors that walk the talk, and stay ahead in the fast-paced world of pharma AI!
Get on the wait list to access this today. Click here.
4. Take our FREE AI for Pharma Assessment
This assessment will score your current leveraging of AI against industry best practice benchmarks, and you’ll receive a report outlining what 4 key areas you can improve on to be successful in transforming your organization or business unit.
Plus receive a free link to our webinar ‘AI in Pharma: Don’t be Left Behind’. Link to assessment here
5. Learn more about AI in Pharma in your own time
We have created an in-depth on-demand training about AI specifically for pharma that translate it into easy understanding of AI and how to apply it in all the different pharma business units — Click here to find out more.