In the fast-paced world of technology, the ‘build fast, fail fast’ approach has become a mantra for many startups and tech giants. This methodology, characterized by rapid development cycles, quick iterations, and a willingness to learn from failures, has fuelled remarkable innovations and breakthroughs.
For instance, companies like Facebook and Google have thrived by embracing this agile mindset, allowing them to pivot and adapt to ever-changing market demands swiftly.
However, when we shift our focus to the pharmaceutical industry, particularly the application of AI, this approach reveals significant risks and challenges. While the allure of rapid innovation is tempting, the stakes in pharma are profoundly higher. The rigorous regulatory environment, the intricate complexity of biological systems, and the paramount importance of patient safety demand a more measured and cautious approach.
In this context, the ‘build fast, fail fast’ philosophy, which may lead to rushed development and insufficient testing, can result in dire consequences including costly failures, compromised patient safety, and ethical breaches. Thus, while this methodology has undoubtedly driven innovation in tech, its application in AI within the pharmaceutical industry necessitates a re-evaluation to ensure that the advancements in healthcare are both safe and effective.
The Unique Challenges of AI in Pharma
The application of AI in the pharmaceutical industry faces multifaceted challenges, primarily due to the inherent complexity of drug development, stringent regulatory compliance, and critical ethical considerations.
The drug development process involves several detailed steps, including target identification, lead compound discovery, preclinical testing, clinical trials (Phases I-III), and finally, regulatory approval and post-market surveillance. This entire journey can take over a decade and costs upwards of $2.6 billion per drug, with a success rate of less than 12% from Phase I to approval.
Regulatory compliance is another significant hurdle. Bodies like the FDA in the United States and EMA in Europe impose rigorous requirements to ensure the safety and efficacy of new drugs. These include extensive documentation, rigorous clinical trial data, and a comprehensive review process. AI applications must navigate these stringent standards, often facing challenges such as the need for transparency in AI decision-making processes and the validation of AI models against real-world data. For instance, IBM’s Watson for Oncology faced criticism for recommending unsafe cancer treatments due to flawed training data.
Ethical considerations also play a critical role in the deployment of AI in pharma. The use of AI must ensure patient safety, data privacy, and equitable access to treatments. Moreover, the lack of interpretability in some AI models can hinder trust and adoption. The “black box” nature of some deep learning approaches makes it difficult to understand how they arrive at decisions, which is problematic in a field where transparency is crucial. Efforts to develop explainable AI (XAI) are ongoing, but challenges remain.
Complexity of Pharmaceutical Data
The complexity of pharmaceutical data is multifaceted, encompassing the vast volume and variety of data types, along with the critical importance of data quality and integrity. In the pharmaceutical industry, data comes from numerous sources, including clinical trials, genomic studies, electronic health records (EHRs), and real-world evidence. Each of these data types contributes unique insights essential for drug development.
For instance, a typical phase III clinical trial can generate between 1 and 2 million data points per study, while the integration of genomic data adds another layer of complexity, with the human genome alone comprising over 3 billion base pairs that need to be analyzed.
The sheer volume of data handled in drug development is staggering. It is estimated that the global pharmaceutical industry generates over 2.5 quintillion bytes of data daily. However, the value of this data hinges on its quality and integrity. Accurate, clean data is crucial for ensuring reliable outcomes in drug development. Poor data quality can lead to erroneous conclusions, resource wastage, and, most critically, patient harm. A stark example of this is the Vioxx case, where data integrity issues contributed to the underestimation of cardiovascular risks, ultimately leading to the withdrawal of the drug and significant legal and financial repercussions for Merck.
Overcoming these data challenges requires pharma companies to invest heavily in data governance, standardization, and curation. Techniques like data mapping, data cleaning, and semantic harmonization are crucial for preparing data for reliable AI analysis. Collaboration between data scientists, clinicians, and regulators is also key to ensuring AI in pharma is built on a solid data foundation.
The Cost of Failure in Pharma
The cost of failure in the pharmaceutical industry is staggeringly high compared to other sectors like technology. Bringing a new drug to market is estimated to cost an average of $2.6 billion, including expenses from initial research and development through extensive clinical trials. In contrast, failed tech projects, while costly, rarely approach the billion-dollar losses seen in pharma. For example, Google’s high-profile augmented reality project, Google Glass, was discontinued after just two years, with losses estimated in the tens of millions rather than billions.
The lengthy timelines involved in drug development further compound the financial impact of failures. It often takes 10-15 years for a new drug to progress from discovery to regulatory approval. This means a failed drug candidate can set back research and development by over a decade, significantly delaying potential treatments for patients in need. The Alzheimer’s drug solanezumab, for instance, failed in late-stage clinical trials in 2016 after 15 years in development, leaving Eli Lilly with losses of over $1 billion.
Beyond financial costs, failures in pharma can severely damage public trust and a company’s reputation. The pharmaceutical industry relies heavily on maintaining confidence among patients, healthcare providers, and regulators. High-profile failures, such as the withdrawal of the pain medication Vioxx in 2004 after it was linked to increased risks of heart attacks and strokes, can erode this trust. Merck, the maker of Vioxx, saw its market value plunge by $27 billion in the aftermath as it faced a wave of lawsuits and investigations.
These examples underscore the unique challenges and high stakes of failures in the pharmaceutical industry compared to other sectors. Rigorous testing, robust quality control, and transparent communication are essential to mitigate these risks and maintain the trust that is vital for pharma’s ability to deliver life-saving treatments.
Importance of Rigorous Testing and Validation in AI for Pharma
The allure of rapid innovation in the pharmaceutical sector, particularly with the integration of AI technologies, is immense. However, the temptation to expedite the development process can have severe repercussions. Rushing through the stages of development and validation can result in inadequately tested AI algorithms, which poses significant risks.
The Perils of Inadequate Testing: The use of insufficiently tested AI algorithms in drug discovery and development can lead to false positives or false negatives, with potentially life-threatening implications. False positives may result in the pursuit of ineffective or even harmful drug candidates, wasting valuable resources and exposing patients to unnecessary risks. On the other hand, false negatives can cause promising drug candidates to be overlooked, denying patients access to potentially life-saving treatments. A study published in the Journal of Chemical Information and Modelling highlighted that even small errors in AI models can propagate and amplify across the drug discovery pipeline, emphasizing the need for rigorous testing and validation.
The Human Cost of AI Failures: The impact of AI failures in the pharmaceutical industry extends beyond financial losses; it directly affects patient safety and well-being. Incorrect predictions or recommendations from AI systems can lead to misdiagnosis, improper treatment, and adverse drug reactions. According to a report by the World Health Organization, adverse drug reactions are a leading cause of morbidity and mortality, accounting for approximately 3.6% of hospital admissions globally. The use of thoroughly tested and validated AI algorithms is crucial to minimize these risks and ensure the delivery of safe and effective healthcare interventions.
Building Trust through Rigorous Validation: Rigorous validation processes are essential to establish trust in AI-driven pharmaceutical solutions. Validation involves evaluating the performance, robustness, and reliability of AI models across diverse datasets and scenarios. It helps identify potential biases, limitations, and uncertainties associated with the algorithms. The FDA has emphasized the importance of validation in its guidance on “Computer Software Assurance for Production and Quality System Software,” highlighting the need for comprehensive testing and documentation. By subjecting AI algorithms to stringent validation protocols, pharmaceutical companies can demonstrate their commitment to patient safety and build confidence among healthcare providers and regulatory authorities.
To ensure the reliable and responsible deployment of AI in the pharmaceutical industry, a comprehensive validation framework is necessary.
This framework should encompass multiple dimensions, including:
● Data Validation: Ensuring the quality, diversity, and representativeness of the training data used to develop AI algorithms.
● Algorithm Validation: Assessing the performance, robustness, and generalizability of AI models across different datasets and scenarios.
● Clinical Validation: Evaluating the clinical relevance and impact of AI predictions through well-designed clinical trials and real-world evidence studies.
● Regulatory Validation: Engaging with regulatory bodies to establish guidelines and standards for the validation and approval of AI-based pharmaceutical solutions.
By prioritizing thorough testing and validation, the pharmaceutical industry can harness the power of AI while mitigating risks and ensuring the delivery of safe and effective healthcare solutions. It is essential to strike a balance between innovation and caution, recognizing that the ultimate goal is to improve patient outcomes and advance medical science responsibly.
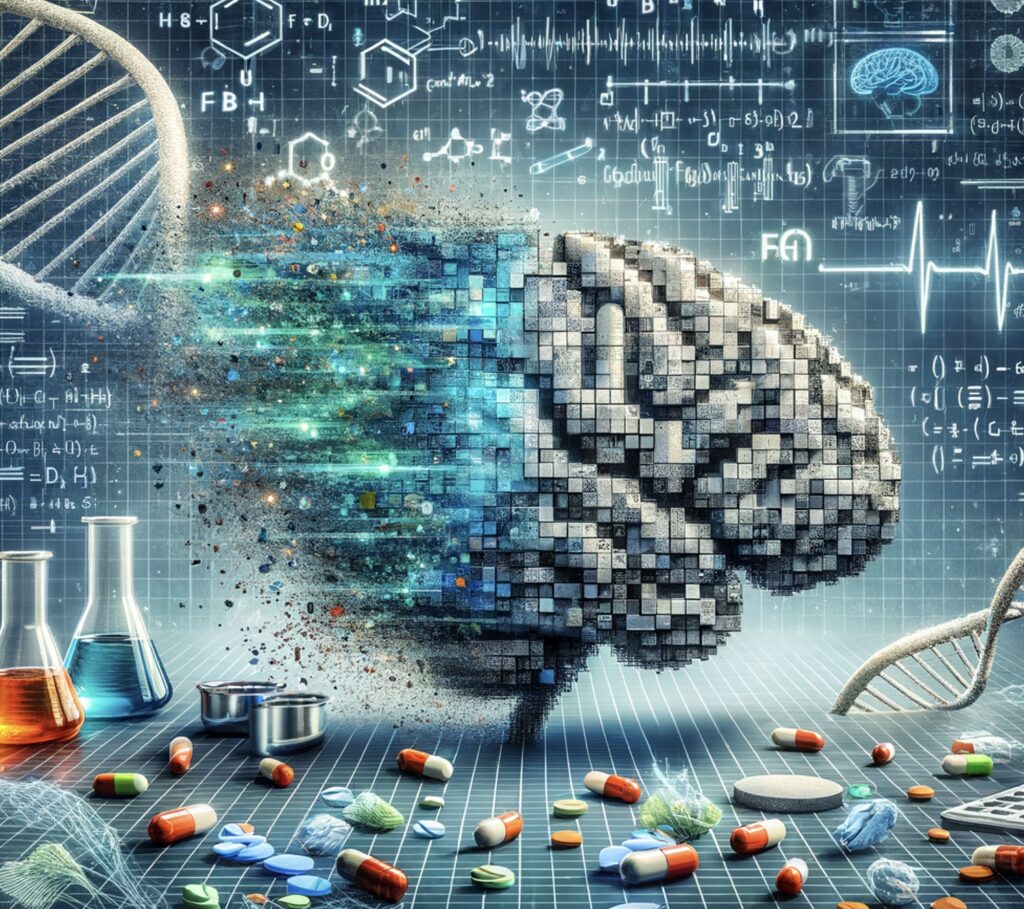
The Need for Robust Data and Ethical Considerations
The development of artificial intelligence (AI) in the pharmaceutical industry hinges on the availability of high-quality data. AI algorithms rely on large datasets to learn patterns, make predictions, and provide insights that can revolutionize drug discovery and personalized medicine. However, obtaining accurate and representative datasets in the pharma sector presents several challenges:
● Data Fragmentation and Siloed Information: Pharmaceutical data often resides in disparate systems and formats. Clinical trial data, electronic health records, genomic data, and real-world evidence are stored across various institutions and platforms, creating significant hurdles for data integration and analysis. According to a study published in Nature Medicine, up to 80% of clinical data remains unstructured and siloed, limiting its accessibility and usability for AI applications.
● Limited Public Availability of Data: The availability of comprehensive datasets is further constrained by the proprietary nature of pharmaceutical research. Much of the data generated in clinical trials and drug development is held by private companies and is not readily accessible to the broader research community. A review in the Journal of Clinical Oncology found that only a small fraction of clinical trial data is publicly available, posing challenges to the diversity and inclusivity of AI training data.
● Data Quality and Completeness: The quality of data used to train AI algorithms is crucial for the accuracy and reliability of their outputs. Incomplete or erroneous data can lead to misleading results and flawed decision-making. For instance, a study in The Lancet highlighted that missing data in clinical trials can significantly bias the findings, impacting the efficacy and safety assessments of new drugs.
The integration of AI in the pharmaceutical industry not only requires robust data but also demands adherence to ethical standards to ensure responsible and equitable use.
Key ethical considerations include:
● Privacy, Transparency, and Consent: Protecting patient privacy is paramount when handling sensitive health data. AI developers must ensure that data collection and usage comply with regulations such as the General Data Protection Regulation (GDPR) in the EU and the Health Insurance Portability and Accountability Act (HIPAA) in the U.S. Transparency in AI processes is also essential to build trust among stakeholders. Patients should be informed about how their data is used, and explicit consent must be obtained.
● Addressing Biases and Ensuring Fairness: AI algorithms are only as good as the data they are trained on. Biased or unrepresentative data can perpetuate and even exacerbate existing health disparities. To mitigate such risks, it is crucial to ensure that AI training data is diverse and representative of the entire patient population. This involves actively seeking out and including data from underrepresented groups and continuously monitoring AI systems for unintended biases.
By prioritizing high-quality data and adhering to ethical principles, the pharmaceutical industry can harness the transformative potential of AI while safeguarding patient rights and promoting equitable healthcare outcomes.
Alternative Approaches Used in Pharma
Exploring alternative approaches in the pharmaceutical industry is crucial to overcoming the limitations of the “Build Fast, Fail Fast” methodology. Pharma companies are exploring alternative approaches, such as incremental innovation, collaborative development, and regulatory sandboxes. These approaches can also be applied to leveraging AI in pharma.
Incremental innovation involves making smaller, iterative improvements to existing drugs or processes. This more measured approach allows for a better balance between innovation and risk mitigation. For example, the development of extended-release formulations, like Pfizer’s Xeljanz XR for rheumatoid arthritis, has provided patients with more convenient dosing options while building upon an already proven drug.
Collaborative development is another promising avenue, with partnerships between AI companies and pharmaceutical firms enabling the pooling of resources and expertise. Successful collaborations, such as the partnership between GlaxoSmithKline and AI startup Exscientia, which led to the discovery of a new drug candidate for obsessive-compulsive disorder in just 12 months, demonstrate the potential of these alliances.
Regulatory sandboxes, which provide a controlled environment for testing new technologies or approaches under the supervision of regulators, could also be adapted for the pharma industry. These sandboxes have been successfully implemented in the financial sector, allowing for experimentation with innovative products and services while ensuring consumer protection (FCA). By applying a similar model to pharmaceutical development, companies should test new AI-driven approaches in a secure, controlled setting, potentially accelerating innovation while maintaining the necessary safety and regulatory standards.
Conclusion
The integration of AI in the pharmaceutical industry presents a transformative opportunity, but it must be approached with caution and collaboration. The critical importance of accuracy, stringent regulatory requirements, and complex biomedical data necessitate a measured approach to AI development in pharma.
Rushing the process or deploying inadequately tested algorithms can have severe consequences, jeopardizing patient safety and undermining trust. Rigorous validation frameworks, encompassing diverse data, regulatory compliance, and continuous monitoring, are essential to mitigate risks and ensure reliable AI solutions.
Success in this domain requires interdisciplinary collaboration among AI experts, pharmaceutical researchers, healthcare professionals, and regulatory authorities. By fostering a culture of responsible innovation, the industry can harness AI’s potential to accelerate drug discovery, optimize clinical trials, and deliver personalized treatments while prioritizing patient well-being.
The journey of AI in pharma is a marathon, not a sprint. It demands a long-term vision, commitment to ethics, and willingness to learn. As we navigate this frontier, let us remember that the ultimate goal is to advance medical science and alleviate human suffering, upholding the highest standards of safety, efficacy, and compassion.
Found this article interesting?
P.S. Here are 5 ways we can help you accelerate your Pharma AI results:
1. Follow Dr Andrée Bates LinkedIn Profile Now
Dr Bates posts regularly about AI in Pharma so if you follow her you will get even more insights.
2. Listen to our AI for Pharma Growth Podcast
Revolutionize your team’s AI solution vendor choice process and unlock unparalleled efficiency and save millions on poor AI vendor choices that are not meeting your needs! Stop wasting precious time sifting through countless vendors and gain instant access to a curated list of top-tier companies, expertly vetted by leading pharma AI experts.
Every year, we rigorously interview thousands of AI companies that tackle pharma challenges head-on. Our comprehensive evaluations cover whether the solution delivers what is needed, their client results, their AI sophistication, cost-benefit ratio, demos, and more. We provide an exclusive, dynamic database, updated weekly, brimming with the best AI vendors for every business unit and challenge. Plus, our cutting-edge AI technology makes searching it by business unit, challenge, vendors or demo videos and information a breeze.
Discover vendors delivering out-of-the-box AI solutions tailored to your needs.
Identify the best of the best effortlessly.
Anticipate results with confidence.
Transform your AI strategy with our expertly curated vendors that walk the talk, and stay ahead in the fast-paced world of pharma AI!
Get on the wait list to access this today. Click here.
4. Take our NEW and FREE AI for Pharma Assessment
When we analysed the most successful AI in biopharma and their agencies, we found there are very specific strategies that deliver the most consistent results year after year. This assessment is designed to give clarity as to how to achieve a successful outcome from AI.
The first step is to complete this short questionnaire, it will give us the information to assess which process is right for you as a next step.
It’s free and obligation-free, so go ahead and complete it now. Plus receive a free link to our a free AI tools pdf and our 5 day training (30 mins a day) in AI in pharma. Link to assessment here.
5. Learn more about AI in Pharma in your own time
We have created an in-depth on-demand training about AI specifically for pharma that translate it into easy understanding of AI and how to apply it in all the different pharma business units — Click here to find out more.