In part 1 of this series (click here to read) we covered:
- The Importance of AI in Pharma
- The Critical Need for a Strategic AI Blueprint
- The Benefits of a Strategic AI Blueprint
Now we will continue by starting with a look at the risks of implementing AI before you do your strategic blueprint.
The Risks of Implementing AI Solutions Without a Strategic AI Blueprint
The implementation of artificial intelligence (AI) solutions in the pharmaceutical industry is gaining momentum due to its ability to drive efficiency, accuracy, and innovation in various business operations. However, implementing AI solutions without a strategic AI blueprint can lead to several risks and challenges that can jeopardize the organization’s success and reputation.
Some of these risks include:
1. Implementing projects that do not align with strategic goals: One of the risks of implementing AI solutions without a strategic blueprint is the possibility of implementing projects that do not align with the company’s strategic goals. This can lead to wasted investment and resources and lost opportunities for the company. Without a clear plan and direction for AI initiatives, companies may pursue projects that are not relevant or beneficial to their overall business strategy and waste huge amounts of money and time.
To mitigate this risk, it is imperative to gain a comprehensive understanding of the company’s strategic goals and how AI can be effectively harnessed to support them. This necessitates a thorough analysis of the organization’s strategic needs and an evaluation of the potential impact of AI initiatives on various facets of the business. By aligning AI projects with strategic goals, companies can optimize the outcomes of their investments and ensure efficient resource allocation.
2. Wasting resources on ineffective projects: Implementing AI solutions without a well-defined blueprint can result in significant resource wastage on ineffective projects that fail to generate value for the business. This squandering of financial resources can hinder progress and impede the achievement of desired outcomes. While AI holds great potential across the pharmaceutical value chain, a lack of clarity regarding the specific problems to be addressed and the intended goals can lead to aimless and unfocused AI initiatives.
According to a study conducted by Gartner, nearly 85% of AI projects fail to deliver the anticipated benefits. Factors contributing to this failure include ambiguous objectives, inadequate data quality, and a lack of skilled personnel. This emphasizes the criticality of establishing a clear AI blueprint prior to implementing any AI solutions.
To mitigate this risk, companies should undertake a comprehensive analysis encompassing factors such as strategic fit, likelihood of success, cost-benefit considerations, and more. This assessment helps evaluate the potential benefits and risks associated with the AI project before committing resources. Furthermore, it is essential to ensure that the project aligns with the company’s strategic goals and possesses well-defined objectives and measurable metrics for success.
3. Inability to scale pilot AI projects effectively
One of the biggest risks of implementing AI solutions without a proper blueprint is the inability to scale pilot projects effectively. Many companies start by testing AI solutions on a small scale, with a limited budget and a few team members. However, if these pilot projects are successful, the company may want to expand its implementation to a larger scale. If the company does not have a solid plan in place for scaling up, the project can quickly become too complex and difficult to manage.
One example of the challenges of scaling up AI projects comes from a study published in the Journal of Medical Systems found that many healthcare organizations struggle to scale AI initiatives beyond pilot projects. The study cites factors such as a lack of infrastructure, limited budget and resources, and difficulty integrating AI with existing systems as common barriers to scaling up.
Without a proper strategic blueprint, a company may also struggle to ensure that its AI solutions are scalable across different regions and markets. Each market may have unique regulatory requirements, cultural differences, and infrastructure challenges that need to be addressed. Failure to account for these factors can result in a lack of adoption or even outright failure of the project.
To avoid this risk, it is essential to incorporate scalability considerations into the AI blueprint from the outset. This ensures that the pilot projects are designed to accommodate the increasing data and complexity as the system scales, resulting in a smoother and more effective scaling process.
4. Failure to foresee strategic risks and implications of AI solutions
When implementing AI solutions without a proper blueprint, it’s easy to overlook the strategic risks and implications of these technologies. For example, the use of AI in healthcare can bring significant benefits, such as increased efficiency and accuracy in diagnosis and treatment. However, it also raises concerns about data privacy, security, and ethical considerations related to the use of patient data.
One notable example of the potential risks of AI in healthcare is the case of Google’s DeepMind and the Royal Free Hospital in London. In 2016, DeepMind was granted access to the personal data of 1.6 million patients without their explicit consent for a kidney monitoring app. The UK Information Commissioner’s Office found that the Royal Free had failed to comply with the Data Protection Act, highlighting the importance of ethical considerations and regulatory compliance when implementing AI solutions.
Another example is the use of biased data in AI algorithms, which can perpetuate systemic inequalities and discrimination. For instance, a study by the American Medical Association found that some popular medical algorithms used to predict healthcare needs were less accurate for Black patients than for white patients, potentially resulting in misdiagnosis or inadequate care. Biases in data can be amplified if not dealt with before starting.
Without a proper blueprint for AI implementation, companies may fail to identify and address these strategic risks, leading to negative consequences for both the company and its stakeholders.
5. Data privacy and security risks
The implementation of AI solutions without a well-defined blueprint raises significant concerns regarding data privacy and security within the pharmaceutical industry. Pharmaceutical companies handle vast quantities of sensitive data, encompassing patient information, clinical trial outcomes, and proprietary research data. The potential consequences of this information falling into the wrong hands are severe, including legal and financial ramifications, damage to the company’s reputation, and potential harm to patients.
Examples of data breaches and privacy violations in the pharmaceutical industry are not uncommon. In 2019, an unsecured server containing the personal data of over 8 million patients was discovered, which included information such as their names, addresses, dates of birth, and medical histories.
In 2020, a major pharmaceutical company disclosed that it had experienced a data breach that potentially exposed the personal and medical information of 300,000 patients.
Another notable incident took place in 2015 when Anthem Inc., one of the largest health insurers in the United States, fell victim to a cyberattack, leading to the exposure of personal information for 78.8 million individuals. This breach resulted in the company incurring over $115 million in settlement fees and associated costs.
AI solutions that utilize large amounts of sensitive data can also be vulnerable to cyber attacks, which can be costly and disruptive. To mitigate these risks, pharmaceutical companies must implement rigorous data protection and security protocols and ensure that they are compliant with data privacy regulations such as the General Data Protection Regulation (GDPR) and the Health Insurance Portability and Accountability Act (HIPAA).
Furthermore, it is imperative for pharmaceutical companies to uphold patient privacy and confidentiality when implementing AI solutions. This necessitates obtaining appropriate informed consent for the utilization of patient data and safeguarding patient anonymity throughout any data analysis processes. Failing to adhere to these principles can result in significant legal and reputational repercussions for the organization.
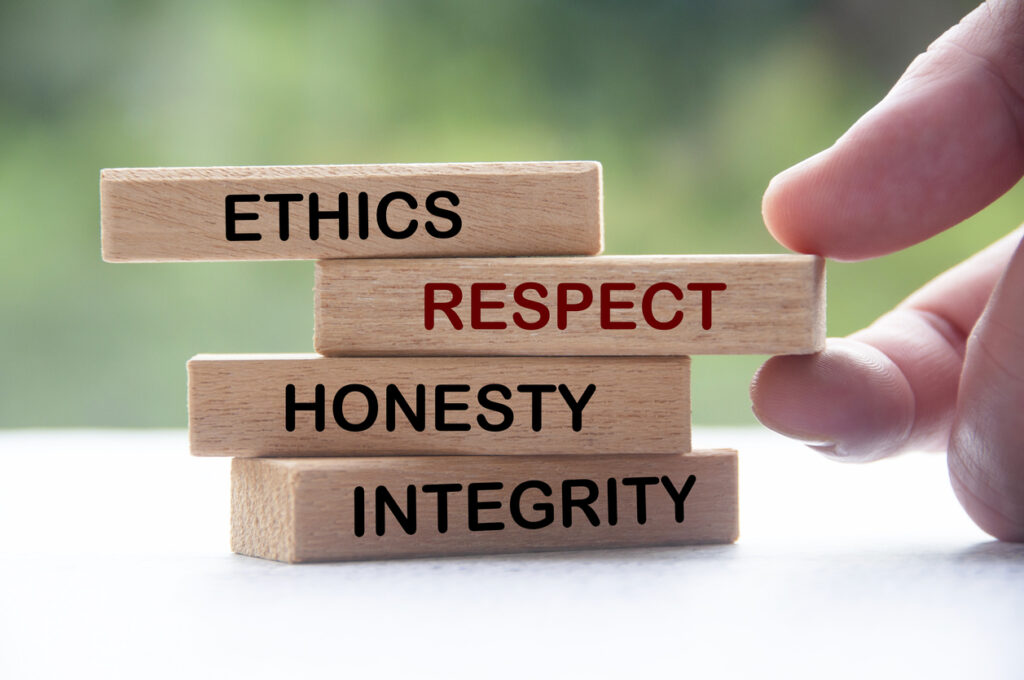
6. Ethical Considerations and Implications
One of the main concerns is the potential for bias in AI algorithms, which could result in discrimination against certain groups of patients. For example, a study found that an algorithm used by some hospitals to predict which patients would need extra care prioritized white patients over black patients.
Another ethical concern is the potential for AI to be used to make life-or-death decisions without sufficient human oversight. While AI may be able to process large amounts of data and identify potential health issues more quickly than humans, it is still not capable of making ethical judgments or taking into account individual patient values and preferences.
Moreover, there are concerns about transparency and accountability, as AI systems are often complex and difficult to interpret, making it challenging to understand how decisions are being made.
Additionally, the use of AI in healthcare raises concerns about the security and privacy of patient data. AI algorithms rely on large amounts of data to learn and make predictions, but this data must be protected to ensure data privacy. As more healthcare data is collected and analyzed, there is an increased risk of data breaches and cyber-attacks.
One example of the ethical implications of AI in healthcare is the use of facial recognition technology to identify patients. While this technology may make it easier for healthcare providers to access patient data and provide more personalized care, it also raises concerns about privacy and potential misuse of the data.
7. Regulatory compliance issues
Implementing AI solutions without a proper blueprint can also result in regulatory compliance issues for companies. The use of AI in the healthcare industry, including pharma, is subject to numerous regulatory requirements and guidelines. Failure to comply with these regulations can lead to legal and financial repercussions for the company.
For example, in the United States, the FDA has issued guidelines for the development and use of AI in medical devices. Failure to adhere to these guidelines can result in fines or even product recalls.
Furthermore, compliance with regulations such as the General Data Protection Regulation (GDPR) and the Health Insurance Portability and Accountability Act (HIPAA) is crucial for protecting patient data privacy. AI solutions that handle patient data must be designed with compliance in mind to avoid potential legal and financial consequences.
For instance, in 2019, Google was fined $1.7 billion by the European Union for violating antitrust laws with its advertising practices. The company’s use of AI in advertising was deemed to have given it an unfair advantage over its competitors. This example shows how regulatory compliance issues can arise even for established companies like Google, emphasizing the importance of implementing AI solutions with compliance in mind from the outset. Pharma companies are subject to far stricter regulations than companies such as Google so it is imperative that we adhere to regulations compliantly.
8. Unforeseen consequences and risks
The implementation of AI solutions without a blueprint can also lead to unforeseen consequences and risks. One of the major risks is the lack of transparency and interpretability of the algorithms used in AI systems, which can lead to unintended outcomes.
For example, in healthcare, if an AI system is trained on biased data, it could lead to incorrect diagnoses or treatment recommendations, resulting in harm to patients.
Another risk is the potential for AI systems to amplify existing inequalities. For instance, AI systems used in hiring or lending decisions may replicate existing biases and perpetuate discrimination against certain groups of people. This can have significant legal and reputational implications for organizations.
Additionally, there is a risk of over-reliance on AI systems, leading to a loss of human judgment and decision-making. This can be particularly problematic in critical domains such as healthcare, where decisions made by AI systems could have life-or-death consequences.
It is crucial to thoroughly evaluate and monitor the impact of AI solutions to identify and mitigate any unforeseen consequences and risks. This can be done by implementing ethical guidelines, involving diverse stakeholders in the development process, and regularly auditing AI systems to ensure they are aligned with organizational values and goals.
Key Elements of a Strategic AI Blueprint
A strategic AI blueprint is a comprehensive plan that outlines the objectives, strategies, and tactics for implementing AI solutions within an organization. A well-designed blueprint should take into account various factors such as the company’s goals, the available data, resources, and the regulatory and ethical considerations surrounding AI use.
Some key elements of a strategic AI blueprint include
1. Understanding strategic context: Analyzing the corporate and business unit goals and objectives and blockages to achieving these are critical elements of a strategic AI blueprint, as they set the direction for where AI can be leveraged most effectively as an enabler to solve the challenges and meet the objectives. It is important to clearly analyze what the organization can achieve through the use of AI, and how it aligns with the broader business strategy.
For instance, a pharma company may
Have a clear goal where Ai can be leveraged to improve drug discovery and development processes by reducing time and costs, improving efficiency, and increasing success rates. By integrating the corporate or business unit goals and objectives and unmet needs, organizations can focus their efforts and investments on areas that provide the greatest value and help measure the success of their AI initiatives.
To illustrate the importance of setting clear goals, a study by McKinsey & Company found that companies with clear AI strategies and goals outperform those that do not. The study also found that clear goals and a strong business case are critical for obtaining buy-in from stakeholders, including employees, customers, and regulators.
Furthermore, a report by Accenture identified that companies that successfully implemented AI solutions had clear business objectives, which were linked to measurable metrics. They also had a strong understanding of the ROI and impact of AI solutions on their business processes.
2. Understanding stakeholder unmet needs: Understanding stakeholder unmet needs is a crucial element of an AI blueprint. It involves identifying the problems that stakeholders are facing and determining how AI can help address those problems. In the pharma industry, stakeholders could include patients, healthcare providers, regulators, and employees. By understanding their unmet needs, pharma companies can create AI solutions that solutions that clearly support or align with desired outcomes such as operational efficiency or better patient outcomes, enhance operational efficiency, and meet regulatory requirements.
Understanding stakeholder unmet needs can help pharma companies identify new business opportunities and revenue streams. For example, by using AI to analyze patient data, pharma companies can identify new patient populations for their existing drugs or develop new drugs to target unmet medical needs.
3. Ecosystem analysis: Ecosystem analysis is an essential element of an AI blueprint, as it helps to identify the various stakeholders, and systems that may be impacted by the AI implementation. It involves mapping out the different components of the ecosystem, such as suppliers, customers, competitors, regulators, and understanding their interactions and dependencies. This analysis helps to identify potential roadblocks, risks, and opportunities and enables organizations to develop strategies for collaborating with stakeholders and managing potential conflicts.
4. Business needs analysis: Business needs analysis is a critical element of an AI blueprint. It involves a comprehensive review of the organization’s business processes and priorities improvement areas, to identify areas where AI solutions can add the most value. This analysis helps to ensure that AI initiatives are aligned with the overall business strategy and can deliver meaningful results.
For instance, in the pharmaceutical industry, AI-powered solutions can help in drug discovery, clinical trial optimization, personalized medicine, supply chain, medical affairs, regulatory, PV, market access, and right through to sales and marketing. A business needs analysis can identify areas where AI can improve these processes or drive innovation, and assist in solving unmet needs and pain points currently existing.
5. Assess strategic fit and evaluate against set criteria: Assessing strategic fit and evaluating potential AI initiatives against set criteria is a crucial element of developing an AI blueprint. This process ensures that the selected AI projects align with the overall strategic goals and objectives of the pharmaceutical company.
These criteria could include feasibility, scalability, likely impact, urgency, cost-benefit analysis, risks of implementation to name a few.
How Pharma Can Ensure AI is Employed Strategically
In today’s rapidly evolving pharmaceutical landscape, harnessing the power of artificial intelligence (AI) is crucial for staying competitive. However, many organizations make the mistake of viewing AI as a plug-and-play solution with immediate returns, leading to fragmented implementation and limited success.
The strategic deployment of AI requires a holistic approach that encompasses technology, culture, and organizational alignment, and the best place to begin to ensure all aspects will ensure success is the creation of an AI strategic blueprint. We offer comprehensive support across various aspects to ensure a well-planned and executed integration and adoption of technology.
A strategic AI blueprint is the first step in planning the approach to leverage AI is a critical first step. The advantages of this are:
1. The ability to have a blueprint that ensures success and allows the shifting from failed pilots to successful company-wide programs: A common pitfall faced by pharma leaders is struggling to move from AI pilots to companywide programs. It is critical to align AI initiatives with broader organizational priorities, ensuring that the initiatives address not only isolated business issues but also the overarching challenges faced by the entire organization. Through the creation of a strategic AI blueprint, we facilitate the transition from pilot projects to comprehensive AI programs that deliver significant business impact.
2. Anticipating and Overcoming Barriers to Change: Each organization faces unique barriers to AI adoption, which may stem from cultural, structural, or process-related factors. A strategic AI blueprint assists pharma leaders in anticipating these barriers by conducting an understanding of the specific challenges that may hinder adoption.
3. Budgeting: To ensure the successful scaling of AI initiatives, organizations must assess whether there is a strong cost-benefit and if so, allocate sufficient resources for successful implementation. Companies that avoid a strategic blueprint often spend far more time and resources than they should due to a lack of upfront understanding and planning.
4. Balancing Feasibility, Time Investment, and Value: We advise pharma leaders to develop a portfolio of AI initiatives with varying time horizons, considering feasibility, time investment, and expected value. Organizations must prioritize initiatives based on a long-term view and the potential for value creation. By combining quick wins with longer-term projects, organizations can achieve a balance between immediate impact and sustained transformation. Starting with an AI strategic blueprint ensures that AI initiatives align with business objectives and deliver value across different time frames.
5. Oversight and Execution: Effective oversight and execution are critical for the successful deployment of AI.
Conclusion
The success of AI implementation in the pharmaceutical industry relies on the early development of a strategic AI blueprint in collaboration with AI experts. While AI is not a panacea, it can effectively address specific challenges and propel business growth. By prioritizing the right issues and aligning AI initiatives with strategic goals, organizations can harness the power of AI to enhance business health and achieve success.
Found this article interesting?
At Eularis, we are here to ensure that AI and FutureTech underpins your pharma success in the way you anticipate it can, helping you achieve AI and FutureTech maturation and embedding it within your organisational DNA.
Our team at Eularis specializes in designing tailored strategic AI blueprints that align all AI initiatives with an organization’s strategic objectives, resulting in significant impact. Through a comprehensive analysis of company strategies, customer needs, and the broader ecosystem, we identify areas where AI can make a significant impact. We assess core AI requirements, evaluate the compatibility of AI projects with the company’s overall strategy, and deliver a strategic AI blueprint document that serves as a roadmap for success. With Eularis, your pharmaceutical or healthcare business can unlock the potential of AI and drive transformative growth.
We are the leaders in creating future-proof strategic AI blueprints for pharma and can guide you on your journey to creating real impact and success with AI and FutureTech in your discovery, R&D and throughout the biopharma value chain and help identify the optimal strategic approach that moves the needle. Our process ensures that you avoid bias as much as possible, and get through all the IT security, and legal and regulatory hurdles for implementing strategic AI in pharma that creates organizational impact. We also identify optimal vendors and are vendor-agnostic and platform-agnostic with a focus on ensuring you get the best solution to solve your specific strategic challenges. If you have a challenge and you believe there may be a way to solve it with AI but are not sure how, contact us for a strategic assessment.
See more about what we do in this area here.
For more information, contact Dr Andree Bates abates@eularis.com.
Contact Dr Bates on Linkedin here.
Listen to the AI for Pharma Growth Podcast on