The U.S. Food and Drug Administration regulates certain AI/machine learning-based medical devices through its premarket review and approval pathways. Any software or device that utilizes AI or machine learning algorithms to aid in the diagnosis or treatment of disease would fall under the FDA’s purview as a medical device. Today it is not only software and medical devices that are powered by AI going through FDA approval. We now also have AI-designed molecules as well as digital twin and synthetic data control arms in clinical trials going for FDA review. We will cover all of these areas in this article.
To date, the FDA has granted marketing authorization to over two dozen AI/machine learning-based medical devices through three main regulatory pathways – 510(k) clearance, De Novo classification or Premarket Approval (PMA). These approved applications span areas like medical imaging analysis, disease screening tools and virtual assistants.
In this article, we will provide an overview of some of the current state of FDA-approved AI, including statistics on approvals to date, the types of healthcare applications receiving clearance, and insights into the FDA’s evolving approach to regulating this innovative technology.
Background on FDA Regulation of AI/ML
The FDA plays a critical role in protecting public health by ensuring the safety and effectiveness of medical products available in the United States. This includes oversight of medical devices, drugs, biologics and other healthcare products. When it comes to AI and machine learning technologies, the FDA focuses its regulatory purview on systems intended for use in the diagnosis, treatment or prevention of disease. Any software or device that utilizes AI or machine learning algorithms to aid clinicians in making medical assessments or treatment decisions would be subject to FDA regulation as a medical device.
To determine if an AI/ML system meets the definition of a medical device, the FDA considers several factors.
Firstly, is the technology intended to be used for a medical purpose such as screening for disease, aiding diagnosis, or monitoring treatment?
Secondly, does the system pose any risks to patient safety if the algorithms were to provide inaccurate clinical insights?
If the answers to these questions are yes, the AI/ML product would likely be regulated as a Class I, II or III medical device, with higher-risk technologies falling into Class II or III. Once deemed a medical device, manufacturers must then receive FDA marketing authorization through one of three main regulatory pathways – 510(k) clearance, De Novo classification or Premarket Approval (PMA).
The majority of lower to moderate-risk medical devices enter the market via the 510(k) clearance process. To gain 510(k) clearance, manufacturers must demonstrate their product is substantially equivalent to a legally marketed predicate device with a similar intended use. Over 80% of new medical devices are cleared through the 510(k) pathway, which typically takes around 90 days for an FDA decision. However, novel AI/ML technologies often lack direct predicate devices due to their new approach to clinical decision-making. In these cases, companies may pursue the more rigorous De Novo classification process.
Through the De Novo pathway, manufacturers can request their novel, low to moderate-risk medical device be reclassified from Class III into Class I or II. This involves submitting a De Novo application to the FDA including detailed information on clinical testing to establish the new device’s safety and effectiveness for its intended use. While taking longer than 510(k) at around 150 FDA days on average, the De Novo pathway provides a critical regulatory on-ramp for breakthrough AI/ML devices that exceed the limitations of the 510(k) program.
A few examples of AI/ML systems granted marketing authorization via the De Novo pathway include IDx-DR for diabetic retinopathy screening and Anthropic’s Pathfinder for pneumonia detection.
For the highest risk AI/ML medical devices classified as Class III, premarket approval (PMA) is required. The PMA pathway is the most rigorous type of FDA review, typically taking 1-2 years to complete. It involves demonstrating reasonable assurance of a new device’s safety and effectiveness based on comprehensive clinical data. While resource intensive, the PMA process provides a pathway for novel AI/ML technologies that diagnose or treat conditions posing the greatest risk if misdiagnosed or mistreated. Overall, through the tailored application of its 510(k), De Novo and PMA pathways, the FDA aims to facilitate patient access to beneficial AI/ML innovations while upholding standards of safety, effectiveness and transparency.
What Types of AI the FDA is Approving Today
The FDA has granted marketing authorization to 691 (as of October 2023) AI and machine learning-based medical devices since its first approval in 2018. The number of annual approvals has grown significantly in recent years, from just 2 devices receiving clearance in 2018 to 108 (from January to October) in 2023. This rapid increase showcases how the agency and industry have advanced in their understanding and evaluation of artificial intelligence as a medical tool.
The AI systems receiving FDA clearance to date can largely be categorized into three main types – AI assistants, AI imaging tools, AI screening/diagnostic tools. In addition to those, we are now witnessing drugs going for FDA approval that are designed by AI and drugs using synthetic data control arms and digital twin data in clinical trials.
The majority of FDA-approved AI/ML devices to date have been in the area of medical imaging. Approved applications of AI include screening or diagnostic tools focused on conditions like diabetic eye disease, cardiovascular risk, lung cancer, and skin disease. Virtual assistants utilizing AI have also gained authorization to provide clinical decision support and accelerate referral workflows. Looking ahead, experts anticipate more approvals in areas like radiology, pathology, dermatology and screening where high volumes of visual medical data can be analyzed. AI is also showing promise in personalized medicine through applications like treatment optimization, biomarker discovery and predicting drug response. Regulators will continue balancing timely access to transformative AI technologies with ensuring patient safety, effectiveness and algorithm transparency remain top priorities as this exciting field continues to evolve.
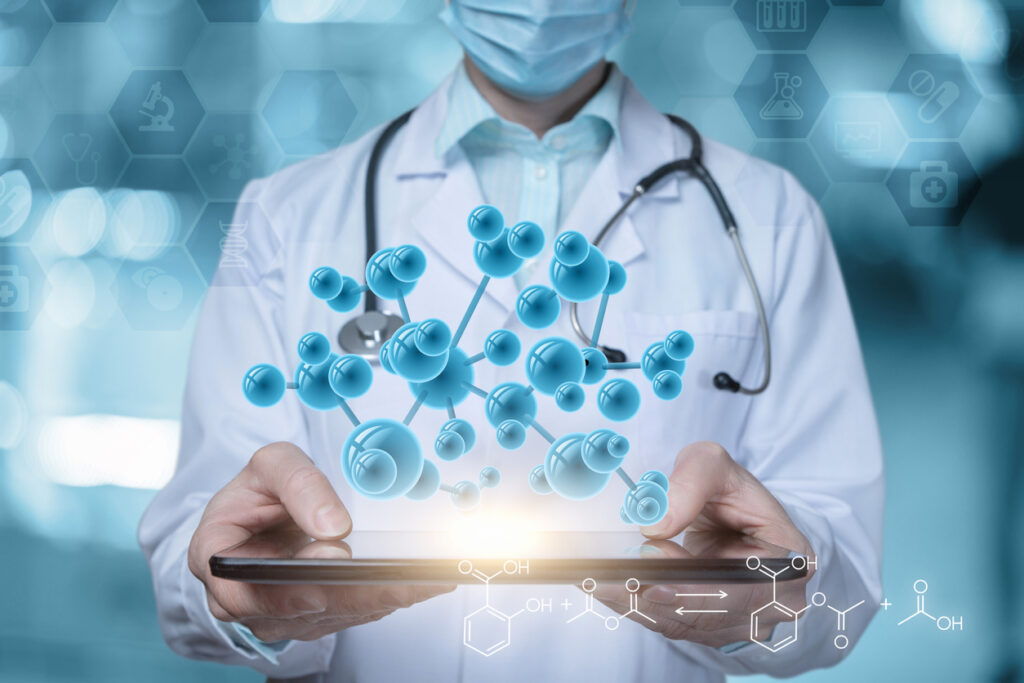
FDA Approved AI-powered Medical Devices
AI assistants are software programs that utilize natural language processing and machine learning to provide clinical decision support and help with referral workflows. Some examples that have been approved include Anthropic’s Claude conversational agent and eMed’s AI assistant. Both of these use natural language processing to answer clinical questions, provide treatment guidelines to practitioners, and help manage patient referral workflows. By supplementing human expertise, AI assistants aim to streamline care delivery and expand access to quality medical advice.
Medical imaging analysis is a major area seeing FDA-approved AI. As previously mentioned, the majority of FDA-approved AI/ML devices to date have been in the area of medical imaging, with over 15 clearances granted for technologies aiding diagnosis or detection from images such as X-rays, CT, MRI and retinal scans. IDx-DR for diabetic retinopathy screening was one of the first imaging tools authorized. Through a randomized controlled trial, IDx-DR demonstrated it could identify the eye condition in patients with diabetes with over 90% sensitivity. The FDA authorized the device via the De Novo pathway in 2018, representing a watershed moment as the agency’s first clearance of an AI-based diagnostic. Another ground-breaking approval was Anthropic’s Pathfinder in 2021, an AI system to analyze chest x-rays and help clinicians detect signs of pneumonia. Pathfinder received a second De Novo authorization, highlighting the potential for AI to improve the accuracy and accessibility of medical imaging analysis. Other FDA-cleared examples include Caption Health’s MammoScreen for breast cancer screening, and Densitas’ AI breast density assessment tool. These are machine learning models that can analyze various types of patient image data like x-rays, CT scans, MRIs and retinal photos to help radiologists and other specialists detect and diagnose conditions.
As radiology and pathology involve vast amounts of visual data, AI is well-suited to efficiently analyze images and flag any abnormalities for human review. Over a dozen FDA-approved devices focus on specialties like radiology, ophthalmology, and cardiology. Going forward, experts anticipate more approvals in specialties like radiology and pathology where AI can efficiently analyze large volumes of visual medical data.
AI screening and diagnostic tools represent a third category receiving FDA marketing authorization. These are software programs trained on patient health records, demographic information, medical images and other data to help identify people who may be at high risk for certain conditions or detect early signs of disease. IDx-DR also falls into this group as its intended use is screening for diabetic eye disease. Other examples include Butterfly Network’s ultrasound tool for foetal abnormalities screening, Anthropic’s lung cancer screening model, and Progenity’s preeclampsia test. Researchers are actively exploring the potential for AI to improve screening accuracy and accessibility across many health issues.
Overall, the FDA’s authorization of these innovative AI-powered technologies demonstrates how machine learning can enhance clinical decision-making across imaging, screening, and healthcare delivery when held to rigorous standards of safety, effectiveness and transparency. Experts anticipate even more transformative AI-based medical applications in the future.
The other areas that FDA has changed their rules for approval are around AI-generated molecules and synthetic data and digital twin data being used in clinical trials.
FDA Approved AI Designed Drugs
While most discussions around FDA-regulated AI focus on medical devices, artificial intelligence is also playing an increasingly important role in early-stage drug discovery and development. Some of the most promising applications of machine learning in pharmaceuticals include generating novel molecule designs, predicting drug properties, assisting with clinical trial design, and identifying new therapeutic targets and biomarkers.
In 2022 there were around 150 small-molecule drugs that had leveraged AI to be designed and of those 15 are already working their way through the clinical trial process.
A prime example is from Exscientia, an AI drug design company that has advanced multiple candidate molecules using their self-supervised AI platform. In 2021, the FDA granted Fast Track designation to Exscientia’s first AI-designed drug, a small molecule for the treatment of certain advanced solid tumors, highlighting the potential of computational approaches.
Another example is from Insilico Medicine has created the world’s first AI-designed anti-fibrotic small molecule inhibitor drug (INS018-055) to be tested in human patients. Distinguishing itself from other AI-driven medications currently undergoing testing, INS018-055 was both discovered and designed using AI. Although notable developments have been observed with INS018-055, only time will tell if it will reach the market. INS018-055 has already completed initial testing and early phases (NCT05154240). In June 2023, Phase II, double-blind, randomized clinical trials began. As of August 2023, the trials are currently being conducted in both the U.S. and China, to investigate the safety, tolerability, pharmacokinetics, and efficacy of INS018_055 administered orally in subjects with idiopathic pulmonary fibrosis (IPF).
Deep learning has shown great potential in generating new molecular structures with optimized properties for potency, selectivity, toxicity and manufacturability – areas traditionally requiring expensive, labour-intensive experimentation. AI drug design platforms can evaluate millions of possibilities in silico to propose candidates most likely to demonstrate efficacy and safety in humans. This represents a paradigm shift from conventional medicinal chemistry techniques.
Other AI innovators like Insilico Medicine, Anthropic, and BenevolentAI are also actively pursuing AI-augmented strategies for developing novel medicines.
AI is also being applied to other drug development tasks like predicting clinical trial outcomes to help with patient stratification and trial protocol design. Analyzing large datasets could reveal biomarkers to identify those most likely to respond or experience adverse events. This type of AI-powered clinical trial optimization aims to accelerate development timelines and improve success rates for bringing new medicines to market.
While AI shows great promise, the FDA is still determining how to regulate AI-designed drugs and ensure their quality, safety and effectiveness and none are fully through to market as of the time of writing. As with devices, transparency into AI models will be important to facilitate scientific exchange and regulatory review. Overall, AI has the potential to revolutionize pharmaceutical R&D, and the FDA’s evolving guidance in this area could help advance this technology’s contributions to public health.
FDA Approved Synthetic Data and Digital Twins in Clinical Trials
AI synthetic data control arms are another area in which companies are receiving FDA approval. A synthetic data control arm is a cohort formed from individuals not enrolled in the focal study but utilized for comparison with a treatment-receiving cohort, with the primary objective of assessing treatment impact. In these trials, the individuals are not actual people but generated AI data made up from generators using real patient data so that they are indistinguishable from real patients data. The data that is used to create the synthetic data in these is either derived from Real World Data (RWD) sourced from hospital and primary care patient records, claims data and mobile apps. The other source of data is the use of historical trial data from patients with the same condition.
One company doing a lot of AI-generated synthetic control arms is MediData who use their database of 9 million+ clinical trial patients to precisely choose matching control and test patients. This method ensures a scientifically valid comparison of the two groups. One recent example of a drug entering Phase III with FDA approval to do so with AI-generated synthetic data is Medicenna’s MDNA55, which uses a highly specific interleukin-4 receptor (IL4R) targeted therapy to treat recurrent Glioblastoma (rGBM).
Digital twin data in clinical trials: Put plainly, a digital twin is essentially a virtual replica of a biological entity, be it a cell, an organ, or even a human being. This digital counterpart enables researchers to observe real-time patient data while also simulating and predicting various clinical outcomes during a clinical trial. Originally stemming from NASA, where computer models simulate the rockets and the space station, the human body presents a far more intricate challenge, lacking a standardized blueprint. Since 2022, the FDA has changed their regulations and will now consider clinical trials with digital twin data. Bayer report that they used digital twin technology to run simulations to inform dose selection for an anticoagulant medication. The data was almost perfectly in line with the results and allowed Bayer to confirm the adequacy of the selected doses. This meant that patients had a lower risk of strokes, heart attacks, thromboses and other undesired side effects. In addition, Bayer were able to predict the level of blood glucose to inform insulin dosing. Bayer has also developed virtual diabetes twins to predict the level of blood glucose that successfully informed insulin dosing. This technology has been instrumental in developing treatments to slow the progression of chronic kidney disease (CKD) and lowers the risk of cardiovascular complications in adults with CKD in type 2 diabetes. Bayer also report replacing control arms in some clinical trials with digital twin data. Bayer has also used digital twins to create virtual trial arms or “external control arms”, which can replace control/placebo arms in some clinical trials. This can help fill evidence gaps e.g., where an RCT (randomized control trial) is not feasible or ethically sound, in addition to reducing costs, overall development time and/or trial recruitment time.
The transformative potential in revolutionizing the landscape of clinical trials from digital twins is very exciting.
Overall, the AI technologies approved to date demonstrate how machine learning can enhance clinical decision-making in areas like medical imaging, screening high-risk populations, and providing timely access to treatment guidelines and specialists. As the technology advances, experts anticipate even more innovative applications of AI to enter the market with the FDA’s oversight helping to ensure safety, effectiveness and transparency remain top priority.
How to Get Your AI Drug Approved
As the use of artificial intelligence in drug discovery and development increases, many companies are seeking clarity on regulatory pathways for AI-designed medicines. While the FDA has approved AI-powered medical devices, the process for novel drugs is more complex given higher safety stakes.
For AI-designed small molecule drugs, the typical approval process would involve first filing an Investigational New Drug (IND) application with the FDA to begin clinical trials. The IND requires information on preclinical data, manufacturing controls, and initial protocols. Companies may meet with FDA regulators early to discuss AI platform validation plans and get feedback on nonclinical plans.
During this stage, the FDA will want to understand how the AI model works at a functional level and see evidence it reliably predicts properties like toxicity, ADME, and potential efficacy in humans. Transparency into model architecture, training data, and performance metrics is key to establishing the AI model’s analytical validity.
If the IND is allowed to proceed, companies then conduct Phase 1, 2 and 3 clinical trials as usual to test safety and efficacy in humans. Here, the AI drug would be evaluated similar to any other investigational medicine. The FDA may ask to periodically re-evaluate any changes to the AI platform over time.
Upon successful clinical trials, the final step is filing a New Drug Application with CMC, nonclinical and clinical data. If approved, any changes to the AI model in the future triggering new drug properties would require further regulatory review. Post-market requirements like adverse event reporting would also apply.
Overall, getting an AI-designed drug approved relies upon rigorous scientific evaluation and human validation at each stage, from model development to clinical testing. With early FDA collaboration, companies can help streamline AI regulatory processes to accelerate access to new medical breakthroughs.
Future Implications of FDA-Approved AI
As artificial intelligence continues advancing healthcare applications, its impact on medicine is poised to grow exponentially in the coming years. More AI and machine learning technologies will likely receive FDA marketing authorization across diverse areas such as medical imaging, disease screening, drug discovery, clinical decision support, and virtual patient monitoring. Regulators will play a key role in facilitating responsible innovation through guidance tailored for AI/ML.
By the end of the decade, analysts project over 50% of new FDA device approvals could incorporate some form of AI or machine learning component. This will drive further expansion of clinical uses like AI-powered endoscopy and pathology systems that can rapidly analyze tissue samples. We may also see AI assist with complex surgeries through applications like robotic control and real-time image analysis.
As AI drug discovery platforms mature, the FDA will likely approve more novel medicines primarily or partially designed in silico as well as drugs that have been tested with digital twin and synthetic data during clinical trials. This could help address areas of high unmet need by accelerating target identification and molecule design processes and speeding up clinical trial timelines. AI may even help develop the first generation of fully personalized medicines tailored to a patient’s unique molecular profile and disease characteristics.
Ensuring the safe, effective, and transparent use of “black-box” algorithms will remain a priority for regulators. The FDA is currently exploring how to assess the analytical and clinical validity of complex AI/ML models without stifling innovation. Areas like model interpretability, performance monitoring, and addressing bias will be increasingly important to facilitate oversight.
If realized responsibly under appropriate oversight, FDA-approved AI has tremendous potential to revolutionize healthcare delivery and outcomes in the decades ahead. Continued collaboration between industry, academia, and regulatory agencies will be pivotal to maximizing AI’s benefits while mitigating risks.
Conclusion
AI is increasingly being leveraged in innovative ways across healthcare to improve patient outcomes and advance medicine. The FDA has authorized at least 691 AI and machine learning-based medical devices since 2018, focusing on areas like medical imaging analysis, disease screening, and clinical decision support. These approvals demonstrate how AI can enhance clinical decision-making when held to rigorous standards of safety, effectiveness and transparency. Moving forward, we will likely see many more AI technologies receive clearance for uses such as personalized disease screening, image diagnostics, drug discovery, and virtual patient care tools.
For AI to reach its full potential, continued collaboration between industry, academia and regulators will be important to maximize benefits, address issues like algorithmic explainability and bias, and help facilitate oversight commensurate with risks. If responsibly guided, FDA-approved applications of artificial intelligence have tremendous potential to revolutionize healthcare delivery and outcomes in the decades to come.
Found this article interesting?
At Eularis, we are here to ensure that AI and FutureTech underpins your pharma success in the way you anticipate it can, helping you achieve AI and FutureTech maturation and embedding it within your organisational DNA.
At Eularis, over 20 years of condensed, tactical, and practical knowledge in pharmaceuticals and healthcare is combined with AI we deliver intensive in-person training in AI for pharmaceutical professionals.
We show you the exact processes, important techniques, and monumental touch points that’ll put you light years ahead of your competition. You will be able to mould, direct, and craft reliable and role-specific AI frameworks – that work every single time!
Register for ‘AI for Pharma Leaders Masterclass’ training to become the leader in your business by strategically using next-generation AI tech to drive superior growth and market dominance. For Pharma and healthcare, it gives you the edge that our industry has been needing for years.
For more information, contact Dr Andree Bates abates@eularis.com.
Contact Dr Bates on Linkedin here.
Listen to the AI for Pharma Growth Podcast on