Imagine a hospital where all the computer systems can talk to each other seamlessly, schedule appointments, and keep track of medical records without human intervention. With the entry of AI-powered multi-agent systems (MAS), that could all be possible sooner than you think.
As a matter of fact, projects like Agent.Hospital and PalliaSys have already laid the groundwork for MAS in healthcare and pharma. AI-powered autonomous agents can help you collect and share medical data from different sources faster, making it easier for patients to access important healthcare information in time.
MAS architectures can enable healthcare and pharmaceutical companies to democratize access to important medical data, improve patient outcomes with predictive analysis, and enable customer satisfaction with personalized medical care at scale.
However, while the potential is almost limitless, you need to tie your technology to strategic business outcomes if you want the best results. Here’s what we think you should know before you start exploring MAS applications within your organization.
What Are Multi-Agent Systems (MAS)?
Multi-agent systems (MAS) are collections of independent autonomous agents that interact with each other within an environment to achieve different goals. These agents can make decisions, learn from their interactions, and adapt to changes in the environment. Each agent in a MAS operates autonomously, possessing its own knowledge base and the capability to perceive, reason, and act within its environment.
Some key features of MAS environments include:
- Autonomy: Agents in a MAS operate independently, making decisions based on their perceptions, knowledge, and objectives without requiring direct human intervention.
- Local Perception: Each agent has a limited view of the entire system. They make decisions based on their local environment and possibly partial knowledge of the state of other agents.
- Decentralization: There is no single point of control in a MAS. Each agent operates independently, contributing to the system’s overall behavior through local interactions.
- Communication: Agents communicate with one another to share information, negotiate, coordinate their actions, and achieve their goals. This communication can be direct (agent-to-agent) or indirect (via the environment).
- Cooperation: Agents often work together to achieve a common goal or optimize a shared outcome, even if they have individual objectives that might not align perfectly.
- Adaptability: Agents can adapt their strategies based on their experiences and changes in the environment.
- Scalability: MAS environments are designed to scale easily, allowing for the addition of more agents to the system to handle increasing complexity or workload.
The Current State of AI in the Pharmaceutical Industry
Over the last few years, AI has emerged as a focal point for R&D initiatives at many pharma companies. So far, it has helped democratize access to medical research, improved clinical trial workflows, and enabled personalized patient care at scale for leading healthcare organizations and pharma businesses.
However, many areas for improvement still remain. A few key limitations of traditional AI that can be addressed with MAS:
- AI systems are often designed for specific tasks with fixed algorithms that struggle to adapt when requirements change or new data becomes available. In the pharmaceutical industry, where research and regulatory environments are constantly evolving, these systems can become obsolete quickly, requiring significant effort and resources to update or replace.
- AI systems often require large, integrated datasets to train and operate effectively. However, data in the pharmaceutical industry is typically scattered across different systems and formats, making it challenging for traditional AI to access and integrate this information for meaningful analysis.
- AI’s approach to problem-solving is generally linear and cannot handle the multi-dimensional challenges common in pharmaceuticals, such as predicting drug interactions or optimizing clinical trial designs. These limitations often result in oversimplified solutions that may not be viable in real-world scenarios.
- AI systems frequently operate in silos, making it difficult to share insights or collaborate across different departments and stakeholders in the pharmaceutical industry. This lack of interoperability can hinder the seamless exchange of information and collaborative research efforts.
- Traditional AI relies on pre-defined models and data, limiting its ability to make decisions in real-time based on new or changing information. In pharma, where timely decisions can significantly impact research outcomes and patient health, this can be a critical shortcoming.
Why Multi-Agent Systems Hold Promise for Pharma
While the current applications of AI in the healthcare and pharmaceutical industry have been nothing short of transformative, there are still many opportunities for improvement.
Multi-agent systems, being smart, collaborative, and decentralized — can help address complex challenges that current technology cannot. Here’s how:
- Efficient Information Sharing: MAS enable different systems within healthcare to communicate effortlessly, allowing for quick access to patient records and making healthcare more efficient.
- Enhanced Coordination: Agents can manage and coordinate healthcare services, such as appointment scheduling and patient care, reducing errors and improving overall service delivery.
- Adaptive and Responsive: MAS are capable of adjusting to new healthcare demands and individual patient needs, offering tailored care experiences.
- Improved Decision-making: By consolidating data from diverse sources, MAS support healthcare professionals in making more informed clinical decisions.
- Resource Optimization: MAS optimize the utilization of healthcare resources, which can lead to reduced waiting times and enhanced accessibility of services.
Unlike traditional AI, which typically focuses on individual tasks or decisions, MAS can manage complex and interrelated processes using multiple agents working in coordination. This enables more flexible, efficient, and scalable solutions, especially in dynamic healthcare settings where information is distributed across systems and stakeholders.
Applications of Multi-Agent Systems in Pharma and Healthcare
There are endless applications for MAS across pharmaceuticals and healthcare. It can be used to optimize medical workflows, enforce quality control, improve patient outcomes, and much more. Here are a few potential applications:
Clinical Trial Patient Recruitment
Multi-agent systems can help automate and streamline the process of recruiting patients for clinical trials. Agents representing patients, physicians, and trial coordinators can communicate and collaborate to match eligible patients with appropriate trials based on specific criteria.
This can reduce manual effort, speed up recruitment, and help trials meet enrollment goals faster.
Distributed Diagnosis and Care Coordination
Multi-agent architectures enable distributed diagnostic reasoning where specialist agents collaborate to arrive at a diagnosis, especially for complex cases.
Agents representing different providers (physicians, labs, pharmacies, etc.) can coordinate care activities, share relevant information, and ensure smooth handoffs and transitions. This improves diagnostic accuracy, care coordination, and health outcomes.
Personalized Treatment Planning
Agents mining data from electronic health records, lab results, imaging, genomic profiles, etc. can piece together a detailed patient model.
Intelligent agents can then utilize this model to generate personalized treatment plans tuned to the individual patient’s characteristics, predicted response, risk factors, and preferences. The plans can dynamically adapt as new data is collected over time.
Optimizing Hospital Workflows
Agent-based systems can model the complex environment and workflows within a hospital. Agents representing patients, providers, departments, equipment, and other resources can simulate “what-if” scenarios.
This can help identify bottlenecks, streamline patient flow, optimize resource utilization, and improve overall operational efficiency. Staff scheduling, bed management, and patient routing can all benefit.
Elderly Home Monitoring
Networks of intelligent agents can enable unobtrusive monitoring of elderly patients in their homes. Wearable and ambient sensor data is continuously analyzed by agents to detect changes in activity levels, medication adherence, vital signs, etc.
The system can alert caregivers and physicians as needed and recommend interventions to keep seniors safe and healthy at home.
Pharmacovigilance and Drug Safety
Multi-agent frameworks can mine real-world data from many sources to identify potential adverse drug events and interactions early.
Agents can correlate data from electronic records, social media, patient forums, research literature, etc. to discover safety signals. Pharma companies and regulators can investigate further, leading to label updates, dose changes, or even drug withdrawal to protect patients.
Epidemic Outbreak Detection
Public health officials can leverage multi-agent systems to detect disease outbreaks early before they spread widely. Agents can monitor heterogeneous data streams like clinic visits, lab test orders, social media mentions, OTC drug sales, work/school absenteeism, etc.
Anomalous patterns are shared between agents and prompt investigation, enabling rapid response to emerging epidemics.
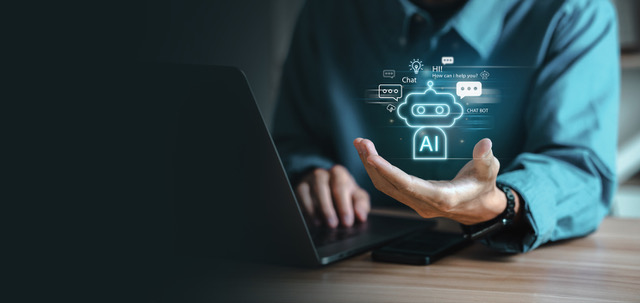
Examples of Multi-Agent Systems Across Pharma
Multi-agent systems are already being experimented on and driving tangible impact across different healthcare organizations and pharma businesses. Let’s understand the potential applications better with a few real-world examples:
TeleCARE
TeleCARE, a project by March Networks and the European Space Agency, uses multi-agent systems to create a supportive environment for the elderly. It’s essentially a configurable framework focused on creating virtual communities to support the elderly, using MAS as the base infrastructure instead of the standard TCP/IP over the Internet.
The underlying infrastructure can be broken down into three tiers:
- External Enabler Level: Supports network communication and interfacing to external devices. Ensures safe data transmission using a VPN.
- Core MAS Platform Level: The main component supporting the creation, launching, reception, and execution of stationary and mobile agents and their interactions. Handles data storage and manipulation. Maintains a catalog of supported devices and services.
- Vertical Services Level: The application level consisting of base horizontal services (web access, virtual community support, specialized interfaces for the elderly) and vertical services that build upon the basic services to enable various interactions.
By combining social interaction, health monitoring, and emergency response through a network of AI agents — the project enables older generations to live safely and independently. Key features of the initiative include automated reminders for medication, detection of abnormal activity patterns indicating potential health issues, and facilitating virtual social interactions to combat loneliness.
PalliaSys
Dealing with a serious illness is an ongoing challenge, not just for the patient but also for their loved ones. PalliaSys uses multi-agent systems to improve palliative care, making sure patients get the support they need, when they need it.
The project is a collaboration between the AI Research Group at Rovira i Virgili University and the Palliative Care Unit of the Hospital de la Santa Creu i Sant Pau in Barcelona. Some of its key aims include:
- Improving the collection of information from palliative patients
- Improving access to this information by patients and doctors
- Helping schedule tasks for palliative care unit doctors
- Monitoring patient state
- Applying intelligent data analysis techniques on palliative care unit data
By using AI agents to handle symptom monitoring, medication management, and communication between care teams, PalliaSys ensures that patients receive timely and coordinated care. It also analyzes patient data in real-time to make adjustments to their care plan as needed.
The project architecture involves a multi-agent system interacting with various IT components like SMS, email, web servers, and database. Then, a data analyzer agent applies data mining and machine learning on the database to generate patient models, predict evolutions, and potentially generate medical protocols.
Another project I uncovered offers a vision of interconnected healthcare services through a MAS framework, facilitating effortless access to medical information and services. The network enables patients to search for medical centers based on specific criteria such as location, expertise, and availability.
Some other key functionalities include:
- Searching for appropriate medical centers based on constraints
- Scheduling appointments with physicians
- Managing medical records with access for both patients and doctors
With a comprehensive medical ontology that standardizes terms and procedures, AgentCities.NET enables clear and effective communication between patients, doctors, and healthcare service providers. This ontology is designed and implemented in RDF to represent knowledge about medical centers, departments, doctors, visits, etc.
Security is a key focus of the solution, with features like encrypted messaging, least privilege access, and PKI-based authentication. The system is based on the FIPA-compliant JADE platform.
Key Considerations for Exploring MAS Applications in Pharma
Multi-agent systems have immense potential to improve AI-enabled healthcare delivery. But to get the most ROI out of your MAS initiatives, combining healthcare domain expertise with agent engineering best practices will be essential.
Here are a few things to keep in mind when exploring potential MAS applications:
Interoperability and Integration
Healthcare environments involve heterogeneous systems, data formats, and communication protocols used by various stakeholders like hospitals, clinics, labs, pharmacies, payers, etc. MAS needs to be designed for seamless interoperability and integration with existing health IT infrastructure, standards (e.g. HL7, FHIR), and workflows. AI agents should be able to access and exchange data securely across system boundaries.
Scalability and Performance
The pharmaceutical industry generates vast amounts of complex and fast-moving data that will only increase as the adoption of electronic health records, wearables, and genomics grows. MAS architectures and communication protocols must be highly scalable to support large numbers of agents, high transaction volumes and low latency interactions. Techniques like mobile agents, dynamic role assignment, and elastic cloud deployments can help.
Security and Privacy
Health data is highly sensitive and heavily regulated (e.g. HIPAA). MAS must implement strong authentication, authorization, encryption, and audit mechanisms to ensure only authorized agent interactions can occur. Agents should have minimal privileges and access data on a need-to-know basis. Blockchain-based approaches are being explored for secure, auditable agent coordination.
Trust and Accountability
In healthcare, lives are at stake and the cost of errors is high. There must be clear accountability and traceability for agent actions and decisions. When intelligent agents exercise autonomy, it’s critical to define decision boundaries, establish human oversight, and maintain the ability to inspect and override agent behaviors. Building trust is key to driving adoption.
Safety and Reliability
In pharma, MAS initiatives are often safety-critical systems — agent failures or unintended behaviors can directly harm patients. Rigorous verification is needed, spanning requirements, design, implementation, and deployment. Agents must be engineered for high reliability and graceful degradation. Formal modeling techniques can help provide safety and liveness guarantees.
Explainability and Transparency
To facilitate human understanding and trust, agent behaviors and decision-making should be as transparent and explainable as possible, especially when machine learning is involved. Techniques like rule-based reasoning, argumentation, and human-interpretable policy specifications can help. Clinicians need to understand the rationale behind agent recommendations.
Ethical Alignment
As agents become more sophisticated, it’s crucial that their goals and behaviors align with human values and ethical principles. Agents may need to reason about privacy, equity, patient autonomy, clinical best practices, etc. when making decisions. Ethical constraints must be elicited from stakeholders and encoded into agent designs. Ongoing monitoring for unintended consequences is important.
Human-Agent Interaction
The socio-technical aspects of how human users interact with and control healthcare agent systems are critical. User interfaces and workflows must be intuitive and tailored to the needs of clinicians, patients, administrators, etc. Careful design is needed to keep humans in the loop, avoid automation surprises, and support effective collaboration. Change management is also key.
Conclusion
Strategy Is the Real Key When Exploring Multi-Agent Systems in Pharma and Healthcare
While multi-agent systems have existed for longer than many of us realize, technological hurdles have prevented their widespread adoption in pharma until recently, aside from a few niche projects. But, with new strides in AI technology being the norm throughout 2023 and 2024, autonomous agent networks are now much more easily accessible to business users.
There has never been a better time to explore and implement MAS in AI-enabled pharma initiatives. However, CEOs and CTOs need to pay close attention so that each project is tied to a strategic outcome, rather than being purely technology-led.
Far too often, we see Fortune 500 companies jumping head-first into new R&D initiatives without a clear strategic goal in mind. This leads to lackluster outcomes, abandoned projects, and sunk costs. That’s why, before you explore applications of multi-agent systems within your organization, you need to have a sound strategic blueprint as a base.
Once you have a clear idea of the operational challenges and bottlenecks within your organization, you’ll know which research initiatives to focus on to make the most impact. You’ll also have a better idea of the resources you can allocate toward these challenges and the kind of return on investment you can expect.
Found this article interesting?
At Eularis, we are here to ensure that AI and FutureTech underpins your pharma success in the way you anticipate it can, helping you achieve AI and FutureTech maturation and embedding it within your organisational DNA.
At Eularis, over 20 years of condensed, tactical, and practical knowledge in pharmaceuticals and healthcare is combined with AI we deliver intensive in-person training in AI for pharmaceutical professionals.
We show you the exact processes, important techniques, and monumental touch points that’ll put you light years ahead of your competition. You will be able to mould, direct, and craft reliable and role-specific AI frameworks – that work every single time!
Register for ‘AI for Pharma Leaders Masterclass’ training to become the leader in your business by strategically using next-generation AI tech to drive superior growth and market dominance. For Pharma and healthcare, it gives you the edge that our industry has been needing for years.
For more information, contact Dr Andree Bates abates@eularis.com.
Contact Dr Bates on Linkedin here.
Listen to the AI for Pharma Growth Podcast on