Discovering and developing new drugs is critical to treating diseases effectively. It’s also an essential part of the pharmaceutical business model, as the commercialisation of new drugs remains a primary growth factor for both large and small pharmaceutical companies.
Current drug discovery and R&D practices, however, are costly, time-consuming and inefficient. In fact, only about 0.1% of candidate molecules make it to market, and only after many thousands of hours of work by hundreds of researchers.
Artificial intelligence (AI), and especially machine learning (ML) offer new ways to approach this problem, and early adopters are already seeing massive improvements regarding costs, time to market, and candidate success rates. When paired with the expertise and experience of its human counterparts, artificial intelligence promises to revolutionise drug discovery and R&D.
In this article, I discuss the imminent need for pharma to transform existing R&D practices and examine some of the ways AI is being used to reduce costs and improve outcomes in drug discovery, treatment development, clinical trials and analysis, and market access.
Why Pharma R&D Needs to Transform
Research and development is a critical component of the pharmaceutical business model. The generation of new drugs is essential to maintaining growth for many pharmaceutical companies, especially as more and more patents reach their expiration date. This is perhaps one of the reasons the number of new drug approvals has increased by 60% compared with the previous decade. Furthermore, of the top 20 largest R&D spenders worldwide, pharmaceutical companies account for nearly half.
However, current R&D practices are extremely costly. Estimates of R&D costs per new medicine range from nearly US$1bn to upwards of US$2.82 and are likely to continue to increase if we continue to rely on traditional methods. Indeed, R&D investments in the pharmaceutical industry have grown at an accelerated rate compared to other industries like semiconductors, software and hardware.
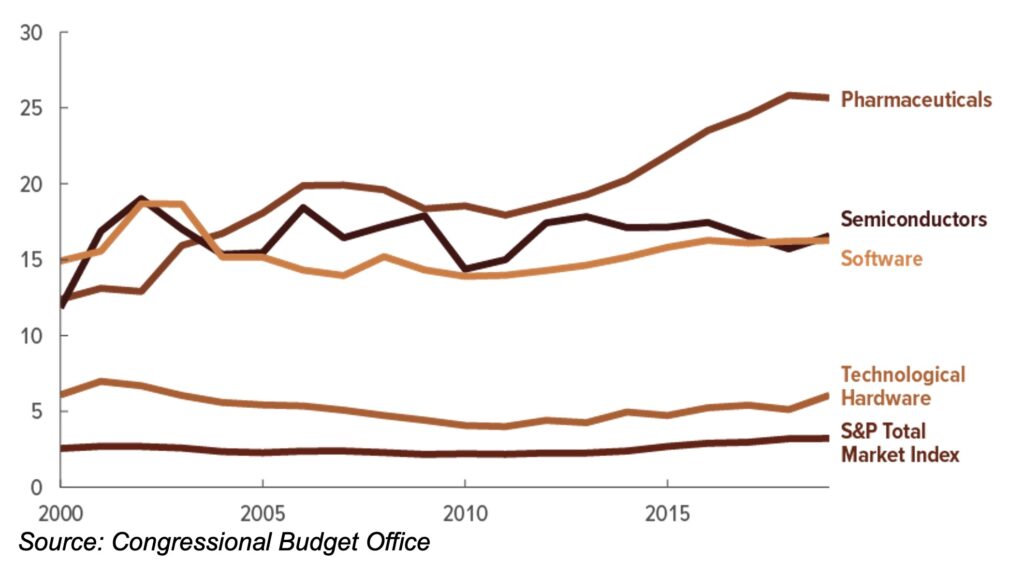
The industry spent nearly US$83 on R&D, about 10 times what was spent just forty years ago.
As the pharmaceutical industry changes, costs elsewhere are very likely to increase, too. The next generation of pharma business models will require significant investments into the health management space. The pressures to reduce costs to drug discovery will be even greater than they are now.
Drug discovery is also a slow and lengthy process. Studies have shown that it takes a new molecule anywhere from seven to 10+ years to go from preclinical phases to market. Data from Roche’s pipeline indicates it takes more than 7 000 000 hours of work and 6 500 experiments to develop a single drug.
Traditional approaches like high throughput testing use ‘brute force’ tactics to wade through millions of candidates, looking for matches with only rudimentary statistical analysis to inform which candidates are most likely to succeed.
Clinical trials can last for years, and the analysis of trial results is both time-consuming and highly complex.
Finally, going to market and meeting regulatory requirements typically adds at least another 8-10 months, and often more.
Thus, while the current approach to R&D has served the industry relatively well in the past, pharma must adopt the use of powerful, new tools to meet the demands of changing markets and continue to find effective treatments to diseases.
How AI can help
Artificial intelligence and machine learning allow us to analyse enormous quantities of data and discover hitherto undiscovered patterns and trends.
By feeding a machine learning algorithm with millions of data points, it can be trained to produce human-like solutions to a variety of problems, such as how to translate a novel, predict the weather, or find new candidates for drug therapies with a high level of success.
Indeed, early adopters are already testing these techniques on the pharma R&D pipeline, with exciting outcomes.
Artificial intelligence is being implemented at every stage of the R&D pipeline, although two arenas stand out in particular: finding candidate molecules and conducting clinical trials.
Finding bioactive compounds
Based on our current knowledge of organic chemistry, the number of possible molecular structures is estimated at 1060. That’s roughly 1000 times the number of atoms in our entire solar system.
It’s commendable, then, that our current practices allow us to take even just 0.1% of candidate molecules to market.
But today’s techniques, such as traditional and even ultra-high throughput, rely predominantly on “brute force” to find molecules. They involve testing millions and millions of candidates to find bioactive molecules that match reduced graphs (rough chemical sketches of what potential candidates may look like).
Fortunately, AI is enabling us to tackle these problems with more finesse. At least 270 companies are currently working on AI-driven drug discovery. Only about 40 of them have their own pipeline, which means that the potential for new partnerships with existing manufacturers is huge.
MIT researchers, for example, successfully used a type of machine learning known as neural networks to rapidly find multiple candidates for antibiotics from millions of molecules. AstraZeneca, in collaboration with BenevolentAI, has identified numerous new targets for treating idiopathic pulmonary fibrosis.
Hypothesis generation tasks in experimental biology have been reduced from weeks to minutes, large molecule structure inference performed up to 100x faster, plate-image screening analysis up to 10x faster with greater accuracy, and high-throughput screening improved in effectiveness by 30-50%.
Clinical trials
Clinical trials take up a significant portion of the R&D budget. High costs, lengthy timelines, difficulties in recruiting and maintaining participants, increasing competition for qualified investigators and sites, and increasingly strict regulatory requirements are all known obstacles to successful clinical trials. Indeed, 85% of clinical trials fail to meet deadlines and more than 10% of sites never enrol a patient.
Thus, the application of AI solutions to address these barriers can have an enormous impact on the cost-effectiveness, speed and success of trials and thus the production of new drugs.
Here are just a few examples of companies doing just this:
1. Zephry AI are ‘building novel transparent AI to address unmet needs across the full spectrum of Precision Medicine from prevention to treatment to improve patients’ lives.’ I recently interviewed the founder and the CEO of Zephyr AI on my podcast ‘AI for Pharma Growth’. You can find the interview here on Apple and here on Spotify.
2. Unlearn recently signed on with Merck KGaA to improve the efficiency and speed of immunology trials. Unlearn’s TwinRCTs™ solution is able to “reduce the size of control arms by 30% or more”, while generating reliable clinical evidence at a much faster rate and greatly reduced cost.
3. Owkin uses machine learning to reduce risk and delays associated with clinical trials. It uses “federated (machine) learning”, a decentralised approach to ML where models are safely trained on multiple data providers, thus increasing predictive accuracy while respecting data ownership and privacy. The approach has been successfully used to identify patients with a high probability of responding well to experimental drugs.
4. Deep Lens is collaborating with Oregon Oncology Specialists to add AI to clinical trial screening and automatically identify qualified patients at the time of their cancer diagnosis, thus accelerated enrolment in clinical trials.
5. AiCure is a clinical trial management platform whose advanced AI can better predict how patients will respond to treatment. It offers real-time monitoring of patient dose behaviour, allowing for smaller, faster trials with more personalised care.
Conclusion
Artificial intelligence is being used up and down the pharma value chain today to great effect. The costly, lengthy, complex process of drug discovery and pharmaceutical R&D can benefit enormously from the application of AI and ML, which promises to reduce costs and delays, improve outcomes and candidate success, and streamline clinical trials. As costs increase elsewhere to meet changing demands from payers, patients and practitioners, the ability to effectively and efficiently develop new drugs will become an even more important factor to success.
Found this article interesting?
At Eularis, we are here to ensure that AI underpins your pharma success in the way you anticipate it can, helping you achieve AI maturation and embedding it within your organisational DNA.
We are the leaders in creating future-proof strategic AI blueprints for pharma and can guide you on your journey to creating real impact and success with AI in your discovery, R&D and throughout the biopharma value chain and help identify the optimal strategic approach that moves the needle. We also identify optimal vendors and are vendor-agnostic and platform-agnostic with a focus on ensuring you get the best solution to solve your specific strategic challengs.
See more about what we do in this area here.
For more information, contact Dr Andree Bates abates@eularis.com.