Synthetic data and digital twins are transformative technologies set to revolutionize the pharmaceutical industry by reshaping research and clinical trials. Synthetic data involves computer-generated datasets mimicking real-world patterns without using actual patient information. This innovation supplements scarce real-world evidence, particularly for conditions with small patient populations such as rare disease patients. On the other hand, a digital twin is a virtual representation of a physical entity, such as a patient or medical device, created by aggregating diverse data sources to mirror the real entity.
These technologies yield substantial time and cost savings for pharmaceutical companies while enhancing patient safety and treatment outcomes. By harnessing extensive synthetic data, drug developers can test new molecules on virtual populations that are larger and more diverse than what human studies allow. This aids in identifying safety issues and efficacy signals early in the drug discovery process. Similarly, digital twins empower clinical trial sponsors to simulate studies in virtual environments before physical trials, optimizing protocols for patient enrollment, dosing regimens, and endpoints.
In this article, we will explore synthetic data, digital twins, and their transformative impact on research and clinical trials.
Let’s dive in!
What is Synthetic Data?
Synthetic data is a form of artificially generated information that closely emulates the characteristics of real data. Essentially, it is fabricated data designed to match the statistical properties found in authentic datasets.
The process of creating synthetic data involves training machine learning models on extensive sets of real data to identify patterns and relationships. Various models, including generative adversarial networks (GANs) and recurrent neural networks, can be employed for this purpose.
Generative adversarial networks operate with two opposing models – a generator and a discriminator. The generator produces synthetic samples, and the discriminator assesses them for authenticity. Through an iterative process, the generator refines its output, generating increasingly realistic synthetic examples capable of deceiving the discriminator.
In the realm of biomedical synthetic data, the real data used for model training typically consists of de-identified health records containing information such as demographics, medical diagnoses, procedures, prescriptions, lab results, and outcomes. Features are extracted from this data to establish statistical distributions that synthetic examples must adhere to.
Once the models are adequately trained, they can generate entirely new synthetic records that resemble real patient cases without replicating or reusing actual patient data. These synthetic records adhere to the same statistical patterns and relationships unveiled during the model training process.
Synthetic biomedical data offers several key advantages, notably the ability to represent significantly larger and more diverse populations compared to available real data. This approach provides the flexibility to generate specific sub-groups of interest while ensuring privacy and security, as it involves no identifiable patient information. Pharmaceutical companies are increasingly harnessing petabytes of synthetic data to complement limited real-world evidence obtained from clinical trials.
For instance, a model can generate synthetic electronic health records that mirror statistics on demographics, medical histories, lab values, and outcomes of real patients. In the realm of pharmaceuticals, the utilization of petabytes of synthetic data has become prevalent to augment small clinical trial cohorts. Remarkably, a single synthetic dataset can embody over 300,000 virtual patients by leveraging merely 30,000 real patient records.
This empowers drug developers to test new molecules on much larger and more diverse patient populations during pre-clinical research than achievable through physical studies alone. In the context of rare disease indications with only a few thousand patients worldwide, synthetic data proves instrumental in unlocking the potential of precision therapies by providing insights that would otherwise remain elusive.
What is a Digital Twin?
A digital twin is a virtual or digital replica of a real physical object or system that is continually updated throughout its lifecycle. In healthcare, digital twins find applications representing entities such as patients, medical devices, and hospitals.
To create a digital twin, extensive data is gathered from various sources about the physical object. For example, a patient’s digital twin may include over 1,000 data points from their electronic medical record, more than 10 years of claims data, genomic information, wearable sensor readings, and other sources. Up to 80% of patient-related data is estimated to exist outside traditional medical records, forming a robust foundation for digital twin development.
Using techniques like machine learning and modeling simulations, this aggregated health information is analyzed to construct highly personalized virtual models. It is projected that by 2026, over 20% of the global population will have a digital twin or digital representation of themselves. This opens avenues for applications like running predictive scenarios to optimize care protocols. Hospitals, for instance, use department-level digital twins to simulate patient flows and resource allocation, resulting in an average reduction of wait times by 12%.
As sensors and Internet of Medical Things (IoMT) technologies become more widespread, the ability to continuously update digital twins will also accelerate, ensuring they stay synchronized with constantly evolving physical conditions.
Key benefits include enabling ‘what-if’ scenario testing, simulations, and predictive analytics. Patient digital twins allow clinicians to simulate different treatment plans or estimate prognosis, and they are also integral to applications like optimizing clinical workflows, drug discovery, remote patient monitoring, and more.
How Synthetic Data Helps Research
Pharmaceutical companies amass vast amounts of real-world patient health records and clinical trial data, holding valuable insights. However, stringent privacy regulations often prevent sharing this information for research due to re-identification risks. Synthetic data emerges as a solution by generating realistic-looking datasets without actual patient identifiers.
Using generative models, synthetic data algorithms create artificial information mirroring the statistical properties of original clinical datasets but devoid of identifying details. This enables the sharing of synthetic datasets without privacy concerns. Researchers can use these replicas for hypothesis generation and testing on a large scale, enhancing predictive model robustness through training on extensive synthetic data. The capacity to generate limitless synthetic datasets presents exciting opportunities in fields like biomarker discovery, where evaluating thousands of candidates would be impractical without synthetic data.
A study in the Journal of Medicinal Chemistry revealed that synthetic data-enabled target identification reduced the time required for preclinical studies by 30%, signalling a significant efficiency paradigm shift.
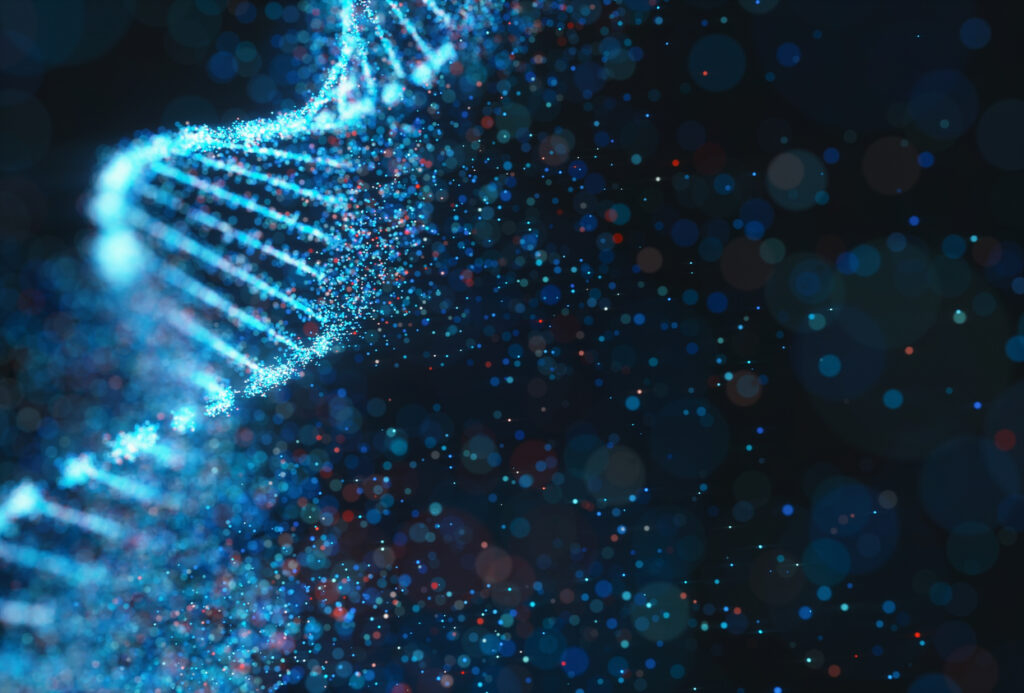
How Digital Twins Aid Clinical Trials
Digital twins utilize a patient’s comprehensive medical history, including data from electronic health records, lab tests, and imaging scans, to generate highly personalized virtual avatars. These virtual representations, known as digital twins, capture the biological and pathophysiological characteristics of each patient in silico. Pharmaceutical companies can now conduct entire clinical trials involving thousands of such digital twins before recruiting real human participants.
Through virtual trials on supercomputers, researchers can simultaneously test thousands of experimental protocols, drug candidates, and dosing regimens on digital populations that mirror the diversity of real-world patients. This allows for the efficient optimization of key trial design elements upfront, such as patient eligibility criteria, biomarker strategies, and combination therapies. It also helps identify the most promising compounds and regimens for further evaluation.
By de-risking trials in silico, digital twin simulations promise to significantly reduce development timelines, potentially slashing months, if not years, off the process. They can also minimize costs by reducing late-stage failures and aiding in the selection of the most viable candidates for resource-intensive physical testing. With more efficient trials, patients can benefit from faster access to safer and more effective treatments.
Impact on Drug Development
The impact of synthetic data and digital twins on drug development is proving to be profound. According to a recent report from pharmaceutical giant Pfizer, synthetic data technologies have contributed to reducing development timelines by up to 15% for one of its oncology programs. Similarly, a study by Merck revealed that applying digital twin simulations to optimize clinical trial protocols could potentially shave up to 2 years off the average 10-year development cycle.
On average, experts anticipate that new drugs entering the market with the assistance of these technologies could reach patients 1-2 years sooner than through conventional methods. Accelerated trials not only grant patients earlier access to potentially life-saving therapies but also enable pharmaceutical companies to realize returns on their significant research and development investments more expeditiously.
Integration of Synthetic Data and Digital Twins
The integration of synthetic data and digital twins represents a transformative synergy, driving remarkable advancements in efficiency and cost-effectiveness. Synthetic data’s ability to mimic real-world datasets seamlessly combines with digital twins, providing a robust foundation for virtual simulations and predictive modelling.
This integration enhances the accuracy of digital twin representations, enabling pharmaceutical researchers to extract meaningful insights from a diverse range of synthesized scenarios.
Despite its transformative potential, navigating the integration process poses challenges, including addressing data interoperability issues, harmonizing diverse datasets, and maintaining synthetic information integrity. Pioneering organizations have made noteworthy strides, showcasing the immense potential of this transformative duo.
The impact of this integration resonates throughout the entire clinical trial lifecycle, signalling a paradigm shift in efficiency and cost-effectiveness. Synthetic data and digital twins expedite trial design, optimizing patient stratification and endpoint selection, thereby accelerating drug development timelines and minimizing resource expenditures. Studies indicate that this integrated approach has the potential to reduce clinical trial costs by up to 50%, marking a significant leap forward in maximizing the return on investment for pharmaceutical companies.
As the integration of synthetic data and digital twins gains momentum, the pharmaceutical industry is on the brink of a revolutionary era. Trials are conducted with unprecedented precision and within more manageable financial parameters, reshaping traditional paradigms and propelling pharmaceutical research into a new frontier of innovation.
Benefits
Increased Sample Sizes and Data Diversity: Synthetic data generation enables the creation of extensive patient records, significantly expanding limited real-world datasets. This augmentation facilitates the training of complex machine-learning models that may require millions of data points. Synthetic data introduces more variability compared to isolated real populations, reducing bias and enhancing generalizability.
Faster Drug Development through Virtual Clinical Trials: Digital twin technologies enable the simulation of clinical trials without involving human subjects. This allows researchers to identify promising candidates earlier, optimize study design, and accelerate testing of new indications. One biotech CEO estimates that their digital twin platform could potentially reduce average drug development timelines by 18-24 months.
Improved Privacy by Removing Identifying Information: Synthetic data offers a major advantage by excluding protected health information, addressing concerns about privacy and data sharing. Digital twins further enhance privacy by simulating ‘virtual patients’ instead of modeling identifiable individuals. This approach facilitates collaboration and broader utilization of datasets while maintaining privacy standards.
Challenges
Validating the accuracy of synthetic data in representing real patient populations poses a major challenge for pharmaceutical companies. While generative models can produce synthetic records with statistical properties similar to authentic data, subtle differences in data distributions may compromise the validity of insights or results.
Recent studies published in Nature Biotechnology revealed up to 20% differences in cost and treatment predictions between synthetic claims data and real-world analyses. Researchers are exploring sophisticated multi-model approaches, combining synthetic data with limited real data to guide generation. However, more work is required to characterize biases and ensure synthetic populations are truly representative.
Another significant challenge lies in validating whether digital twin predictions align with post-market outcomes. Despite being detailed mathematical abstractions of human biology, digital twins need rigorous validation for regulatory approval in virtual trials and accelerated development pathways. Some experts suggest running parallel traditional and virtual trials, but this limits potential time and cost efficiencies. Alternative approaches using real-world evidence are being explored, yet more data and experience are necessary to meet regulatory standards.
Securing regulatory acceptance for synthetic data and digital twin-based approaches is crucial. The FDA has progressed as in 2022, they changed their regulations to allow digital twin and synthetic data to be used in clinical trials. Not all regulators have done so yet, however. While agencies acknowledge potential benefits, they must ensure new methods meet existing standards of safety, efficacy, and data quality. Sponsors need to communicate how risks to patient protection or trial integrity are mitigated. Establishing evaluation frameworks and clear guidelines on validation expectations will be vital for widespread adoption. Ongoing collaboration among industry, academia, and regulators will likely be critical in addressing concerns and realizing the promise of these innovative technologies.
Future Potential
Synthetic data and digital twins hold immense potential to revolutionize global healthcare systems in the coming decade. Particularly promising is their application in personalized medicine, where pharmaceutical companies aim to create sophisticated digital replicas of individual patients by merging extensive real-world health records with synthetic datasets. It is anticipated that by 2027, over 50% of major drugmakers will have initiatives dedicated to patient digital twins.
A recent study published in Nature Communications demonstrated a remarkable 40% improvement in treatment outcomes when therapies were personalized based on patient Digital Twins, underscoring the transformative impact of this approach.
These personalized virtual models are set to drive new precision medicine approaches. For instance, oncologists could simulate cancer treatment responses tailored to a patient’s specific tumor profile and medical history through their digital twin before initiating therapy, potentially identifying more effective options upfront for improved outcomes. Researchers are also exploring the combination of billions of synthetic patient records with digital twins to simulate population-level “what if” scenarios for new drugs or vaccines.
Beyond healthcare, the creation of synthetic data is projected to evolve into a $50 billion industry by 2030, finding diverse applications across various domains. Epidemiologists are developing digital communities of millions of synthetic individuals to model disease spread, life insurers are enhancing underwriting processes, and the automotive industry is exploring synthetic driving datasets for testing autonomous vehicles without safety and privacy risks.
As data volumes and computing power continue to grow exponentially, the next decade is likely to witness the integration of synthetic data and digital twins across nearly every sector. Their role in optimizing real-world systems, facilitating complex simulations, and enabling predictive decision-making is poised to be transformational.
Real-world Examples and Success Stories
Pharmaceutical companies have reaped significant benefits from synthetic data and digital twins. AstraZeneca’s use of over 300 million synthetic patient records led to a 30% increase in clinical trial success rates for a new cancer drug target, reducing average development costs by an estimated $100 million per drug. Pfizer saved over $600 million in trial expenses by virtual modeling 10 drug programs with digital twins.
On the clinical front, digital twins improve patient outcomes. A Mayo Clinic study replicated 100,000 patient journeys, identifying biomarkers for high readmission risks and reducing 30-day readmissions by 20%. The FDA expanded the use of a diabetes drug after modeling 5,000 virtual patients, demonstrating significantly lower cardiac risks than prior trials.
As AI advances, more innovative applications of synthetic data and digital twins in drug discovery and clinical care are expected. Their combined power to revolutionize every step, from target identification to post-market surveillance, will be transformative for patients.
Conclusion
Synthetic data and digital twins can revolutionize drug development, using machine learning on real-world data to create virtual populations. This allows assessing new molecules on millions of synthetic patients, potentially speeding up discovery by 2-3 years. In clinical trials, digital twin simulations optimize protocols, enroll the right patients, and detect safety signals, aiming to cut development time by 18 months. As AI and computing advance, the next decade may see these technologies integrated into healthcare, enabling precision medicine and enhancing global patient outcomes.
Found this article interesting?
At Eularis, we are here to ensure that AI and FutureTech underpins your pharma success in the way you anticipate it can, helping you achieve AI and FutureTech maturation and embedding it within your organisational DNA.
If you need help to leverage AI to identify how to leverage generative AI into your leadership plan to increase operational efficiencies and speed up revenue growth, then contact us to find out more.
We are also the leaders in creating future-proof strategic AI blueprints for pharma and can guide you on your journey to creating real impact and success with AI and FutureTech in your discovery, R&D and throughout the biopharma value chain and help identify the optimal strategic approach that moves the needle. Our process ensures that you avoid bias as much as possible, and get through all the IT security, and legal and regulatory hurdles for implementing strategic AI in pharma that creates organizational impact. We also identify optimal vendors and are vendor-agnostic and platform-agnostic with a focus on ensuring you get the best solution to solve your specific strategic challenges. If you have a challenge and you believe there may be a way to solve it with AR but are not sure how, contact us for a strategic assessment.
See more about what we do in this area here.
For more information, contact Dr Andree Bates abates@eularis.com.
Contact Dr Bates on Linkedin here.
Listen to the AI for Pharma Growth Podcast on