Medical literature monitoring is an essential part of pharmacovigilance (PV). It enables researchers to discover adverse events taking place in the real world, understand the context in which they’ve taken place, and reach important conclusions about drug safety and efficacy.
Traditionally, though, it’s an expensive and time-consuming practice. A pharmaceutical companies’ PV experts may spend hundreds of hours per month pouring over the scientific literature, the vast majority of which is ultimately excluded as irrelevant.
Considering how vast the scientific literature around some diseases already is and the increasing rate at which new studies are released every month, it’s small wonder that the number of valid individual case safety reports (ICSRs) accurately identified and processed is relatively small compared to the volume of articles being published. Efforts to reduce adverse event (AE) under-reporting, currently estimated at 94% worldwide, will only increase the burden on human experts. The situation is critical, for both patients and pharmaceutical companies.
Fortunately, breakthroughs in artificial intelligence (AI)—and especially natural language processing (NLP) and machine learning (ML)—have led to the creation of more sophisticated approaches to medical literature monitoring.
Automated medical literature monitoring (AMLM) can increase the efficiency of experts in identifying and processes ICSRs and AEs by close to 90%, leading to more timely and accurate PV reports, safer conditions and better outcomes for patients, and reduced costs for pharmaceutical companies.
In this article, I explain what AMLM is, how it works and explore the benefits and current challenges of this new technology.
What is automated medical literature monitoring and how does it work?
Automated medical literature monitoring refers to the use of computers to perform some or most of the filtering process and basic analysis involved in medical literature monitoring.
Early, pre-AI attempts at AMLM, by way of, for example, a basic keyword search of article abstracts, showed promise, but were severely limited. Such automation is performed largely without context, resulting in numerous false positives and negatives.
However, AI-based AMLM has proven highly effective indeed. AI AMLM leverages sophisticated algorithms for natural language processing and machine learning to perform many of the basic filtering tasks once executed by human experts—only much, much more quickly.
Human experts are then free to analyse suspected ICSRs and AEs, as determined by the AMLM program, confirming or rejecting the platform’s suggestions. The total number of articles to be evaluated by a human expert is thus reduced from thousands to mere hundreds, or even fewer depending on the drug and disease. The resulting two-tier process, marrying the speed and increasing accuracy of AI with the expertise and experience of human experts, is far more efficient.
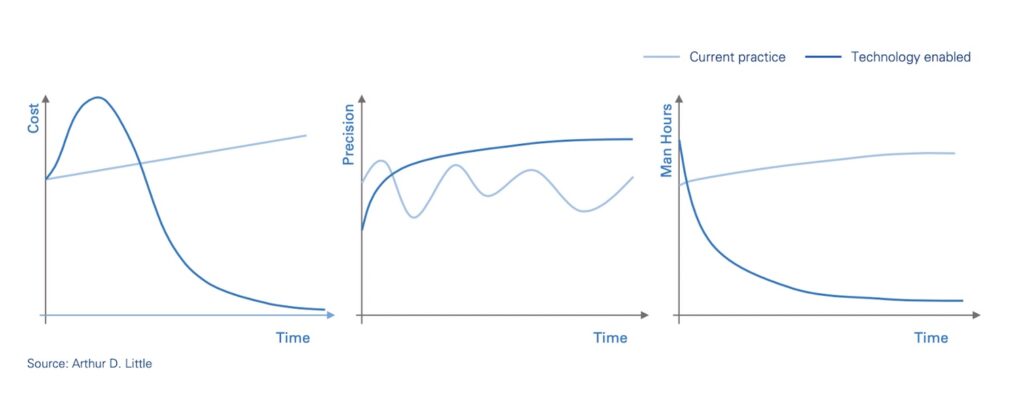
Benefits of AMLM
There are several benefits to using AI-powered AMLM.
Most obviously, it significantly reduces the pressure on human experts to manually review articles, allowing them to focus their attention on those which have the greatest probability of relevance. The potential savings for pharmaceutical companies are significant, approaching 90%. Experts are also less likely to miss critical information or suffer from burn-out—the chances of which will only increase as the literature grows.
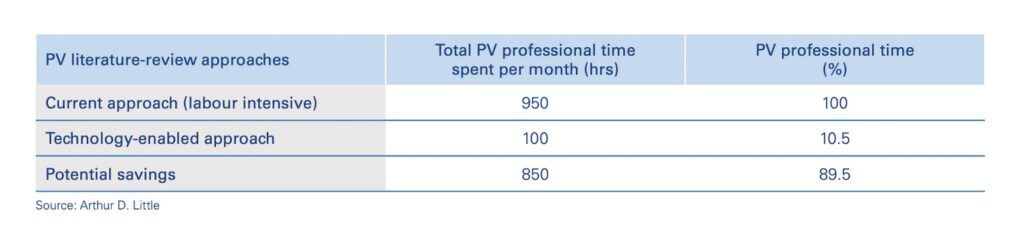
As a result, more AEs and ICSRs can be identified earlier on, resulting in faster and more accurate pharmacovigilance decision-making. Considering the critical importance of PV on patient populations and drug performance, there’s little doubt this will translate to thousands more saved lives and significantly improved business outcomes for pharmaceutical companies, especially as the industry moves towards outcome-based pricing.
The use of natural language generation (NLG) in AMLM also shows promise. Platforms like Sorcero allow for the automated creation of summaries of abstracts, article sections or whole articles. In addition to creating abstracts for studies that lack them (a real hindrance to medical literature monitoring in general), NLG can also be used to create natural-language summaries of specific points of relevance to experts and researchers, ones which the studies’ authors may not have included in the abstract. This saves experts having to skim and skip between article sections to find relevant information, saving time and reducing the risks of missing something critical.
Current hurdles
Of course, as with any new technology, limitations exist that must be taken into account and eventually, as the technology improves, addressed.
Ohano, Sullivan and Beker offer a substantial list of risks and suggested solutions, most of which centre around human oversight at critical points of the process. For example, where “full automation derived from model predictions may hide incorrectly predicted documents from users”, they recommend “diverse levels of supervision and validation”, and avoiding full automation whenever possible.
Other risks come from how machine learning algorithms are trained. “Inconsistency in labelling training data may lead to poor performance”, for example. Likewise, when data used in training “is not representative for the intended use, or is biassed towards certain scenarios”, the platform may provide unexpected or biassed results.
The question of bias in safety reporting and medically-applied AI is not a new one, of course. Such biases range from those related to disease definition and incomplete reporting to, more alarmingly, biases relating to gender and race. Likewise, small differences have been found in industry-funded vs. independent studies vis-à-vis adverse reporting. A machine learning algorithm will “learn” these biases as it is trained on literature. Such biases must be taken into account when training and using AMLM—at the same time as the medical community as a whole does its best to address them at their root, of course.
AMLM beyond pharmacovigilance
Of course, the benefits of AMLM extend far beyond pharmacovigilance. Meta-studies and literature reviews constitute an important part of the scientific process, but the process of selecting, filtering and reviewing research papers is time-consuming and costly, just as it is for safety reporting. Access to simple, powerful, and effective AMLM platforms for research institutions could dramatically increase the prevalence of such reviews while improving their findings.
As mentioned above, AMLM will become increasingly important for pharmaceutical companies as outcome-based pricing becomes more widespread. For outcome-based pricing to be effective and beneficial for patients and pharmaceutical companies, details from clinical trials and real-world evidence must be collected and analysed in a timely fashion.
Indeed, any medical and pharmaceutical workflows that involve a literature review, from the scientific to the commercial, will benefit from access to simple, powerful and effective AMLM platforms.
Conclusion
When paired with the knowledge and industry experience of PV and pharmaceutical experts, Artificial intelligence offers an effective and efficient solution to medical literature monitoring, when paired with. While certain limitations still exist, both technical and cultural, existing AMLM platforms are already being used by early adopters to dramatically increase efficiency and accuracy in pharmacovigilance—an aspect of drug development that is already of utmost medical importance and poised to take on even greater commercial significance.
Found this article interesting?
If you are looking for more insights into how Artificial Intelligence and FutureTech can be strategically applied for automated medical literature monitoring, let us help.
At Eularis, we are here to ensure that AI and FutureTech underpins your pharma success in the way you anticipate it can, helping you achieve AI and FutureTech maturation and embedding it within your organisational DNA.
If you need help to leverage AI to identify how to leverage generative AI into your leadership plan to increase operational efficiencies and speed up revenue growth, then contact us to find out more.
We are also the leaders in creating future-proof strategic AI blueprints for pharma and can guide you on your journey to creating real impact and success with AI and FutureTech in your discovery, R&D and throughout the biopharma value chain and help identify the optimal strategic approach that moves the needle. Our process ensures that you avoid bias as much as possible, and get through all the IT security, and legal and regulatory hurdles for implementing strategic AI in pharma that creates organizational impact. We also identify optimal vendors and are vendor-agnostic and platform-agnostic with a focus on ensuring you get the best solution to solve your specific strategic challenges. If you have a challenge and you believe there may be a way to solve it with AR but are not sure how, contact us for a strategic assessment.
See more about what we do in this area here.
Contact Dr Bates on Linkedin here.
Listen to the AI for Pharma Growth Podcast on
For more information, contact Dr Andree Bates abates@eularis.com.