PharmacoVigilence (PV) is therefore a critical step in the development and commercialisation of pharmaceutical drugs, and absolutely essential in terms of patient safety.
Over time, however, PV has become increasingly more complex. The industry counts more drugs of greater complexity than in previous years. More reports are being generated, with a greater density and variety of information.
At the same time, the stakes are higher than ever before. Expectations from regulators, patients and practitioners are higher and more specific. Patients and practitioners, especially, demand a greater level of personalisation and greater emphasis on preventative medicine and patient support programs (PSPs). New business models have emerged, including value-based and outcome-based pricing models.
Artificial intelligence (AI) and machine learning (ML) stand out as powerful tools to meet these challenges and take advantage of the growing amount of PV data available to pharmaceutical companies.
In this article, I explore several factors driving changes to PV and the challenges and opportunities they present, offer examples of how AI is being used by industry leaders and what obstacles the industry faces, and offer suggestions on how to get started today with artificial intelligence in PharmacoVigilance.
PV in the 21st century: more data, greater complexity
PharmacoVigilance has grown significantly in complexity over the last few years.
The total number of prescriptions being filled has increased by 17% since 2013, and today, the FDA regulates more than 20,000 individual drug products. While some drug regimens have become simplified thanks to combination drugs, like with the treatment of HIV, others have grown in complexity. Advances in drug development and production have resulted in drugs with greater molecular, pharmacodynamic and pharmacokinetic complexity.
As a result, interactions and adverse events have increased in number and complexity themselves, while also becoming more difficult to predict.
At the same time, the total number of adverse event reports (AERs) has increased.
There is more information being generated and more reports submitted than ever before. Importantly, the ways in which this information is presented has also multiplied. Vast amounts of unstructured data related to potential AEs exists in new forms, like social media posts and data collected by wearables.
The industry needs new tools to capture and process this information.
The stakes are higher
In addition to the challenges presented by new types and greater quantities of data, shifting priorities and increased attention from regulatory bodies and the general public have raised the stakes on PV.
Misinformation and confused policies regarding Covid-19 have shaken the public’s faith in the healthcare system and drug companies—despite the industry’s efforts in finding an effective solution to the pandemic. Regulatory scrutiny and pressure has increased as well.
Expectations from practitioners, payers and regulators have shifted significantly over the last several years. There is a much greater focus on patient-centricity, from both commercial and medical perspectives. And the industry itself is moving towards value-based and outcome-based pricing. Successfully navigating this shift will put new demands on PV, whose work is intrinsically tied to patient outcomes and overall drug value.
As a result of this, and in line with general trends over the last two to three decades, costs associated with PV have increased. Indeed, the PV market has increased in value from $7.9b in 2020 to $9.07b in 2022, and is expected to reach $16.23b by 2023. Unsurprisingly, there is significant pressure for companies to find ways to reduce these costs, while still meeting the increasing demands of the public and regulators.
How AI can help meet the challenges of modern PV
If we can meet these challenges, the benefits are manifold. AI and especially ML are powerful tools for capturing and processing AE data.
Dealing with the increase in both quantity and complexity of data, and especially that of unstructured data, is a key challenge. Natural language processors (NLPs) are especially useful across multiple PV stages.
Capturing data from structured and unstructured sources
As mentioned above, the processing and analysis of ICSRs can be especially time-consuming. Advances in Natural Language Processing (NLP) and language-associated ML can help extend the reach and efficacy of PV teams. Regulatory bodies require routine literature monitoring, for example, although this process can be costly, and critical information is sometimes overlooked by human readers. There are some good AI-powered systems that handle these exceptionally well including Reportum by MyMedsandMe which captures data from numerous sources.
Social media presents another opportunity for capturing potential AEs, but its unstructured nature and the presence of colloquial language, not to mention the sheer quantity of information, makes it extremely difficult to analyse without using an AI-powered approach – especially at the intersection of NLP and ML.
Finally, AI can be used to facilitate and simplify the process of AE reporting, both by patients and physicians. In the case of the former, digital humans can be used to provide a more familiar and emotionally comfortable experience for reporting AEs directly to manufacturers, if they are unable or unwilling to do so with their physicians. Regarding the latter, intelligent chatbots can guide physicians through reporting processes in an intelligent fashion, asking contextually relevant questions based on just a few initial pieces of information.
Case intake, coding and processing
Intake, coding and processing is the most costly phase of PV, representing up to 50% of PV spending. Fortunately, this is one area where AI also excels. Machine learning algorithms can be trained on the existing, large quantities of AE data and then used to encode incoming data with industry-altering speed and accuracy. Direct cases are encoded and processed with minimal to no human intervention, freeing human experts to deal with more complex or difficult cases.
To help deal with the more than 1.4 million AEs Pfizer processed in 2019 alone, the company developed its own AI to “handle the more repetitive tasks of AE case report intake and processing.” Pfizer chose to focus its efforts on the intake and processing stages, “where information is received from patients, doctors and others,” freeing up experts to focus on “more of the human interactions and the types of tasks that have the most beneficial impact for patients.”
Data analysis
Of course, once all this information has been collected and encoded, it must be analysed to identify signals and risk factors, identify issues and generate recommendations on drug use. Once again, AI is the best tool for this job. There are end-to-end pharmacovigilance platforms that offer a multitude of AI-based solutions for PV teams. These improve signal detection, simplify compliance, and ultimately allow action sooner.
What this means for the pharmaceutical industry
More data promises more rapid, accurate and broad identification of AEs. This is especially true if we are able to improve processes around individual case study reports (ICSRs). Manual workflows involved in case reporting are time-consuming and costly, and this is where many of the resources allocated to PV are spent. But the integration of AI can help reduce costs across the entire AE reporting process
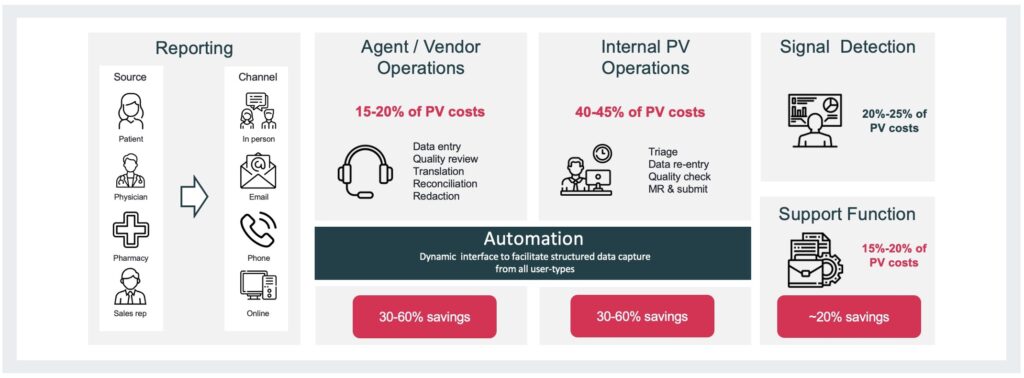
Image courtesy of MyMedsandMe
In addition to reduced costs, the use of AI in PV significantly reduces delays throughout. There are many obvious advantages to this. Harmful drugs can be pulled off the market earlier, saving lives and bolstering trust in drugs that remain on. Our understanding of AEs and the underlying mechanisms will improve, and with it our ability to predict AEs and drug interactions or design drugs and medical devices that reduce or eliminate them.
As our reach grows to include non-traditional sources of AEs, such as social media and online focus groups, we can capture more interactions, and especially interactions with factors beyond the reach of traditional PV, such as socioeconomic factors (which impact e.g. diet), lifestyle (e.g. exercise, stress), and the use of alternative interventions (traditional and holistic medicine, the use of herbs, etc.).
Ultimately, this translates to safer drugs, fewer AEs over time, and fewer hospitalizations. This means better patient outcomes and reduced costs to the healthcare system.
Successful execution of value- and outcome-based business models will also depend on the ability of pharmaceutical companies to capture and analyse AEs in a timely fashion. The financial consequences of failing to do so in such a framework could be catastrophic. This AI-enhanced, extended approach to PV also feeds well into increased demands around patient programs and patient-centric business models, such as “owning a disease.”
How to get started with AI-Driven PharmacoVigilence
As we have seen, there are many ways in which artificial intelligence can be used to improve PharmacoVigilance workflows and mechanisms. Early adopters are already reaping the benefits of doing so, with significantly reduced costs and delays and improved efficacy.
While implementation requires resources and careful planning, the benefits are significant. Furthermore, failing to stay ahead of the curve will mean struggling to manage the requirements of regulators and expectations of patients and practitioners.
Found this article interesting?
To get started transforming PharmacoVigilance data into business decisions today, contact us to discuss your needs and develop a clear path forward.
At Eularis, we are here to ensure that AI and FutureTech underpins your pharma success in the way you anticipate it can, helping you achieve AI and FutureTech maturation and embedding it within your organisational DNA.
If you need help to leverage AI to identify how to leverage generative AI into your leadership plan to increase operational efficiencies and speed up revenue growth, then contact us to find out more.
We are also the leaders in creating future-proof strategic AI blueprints for pharma and can guide you on your journey to creating real impact and success with AI and FutureTech in your discovery, R&D and throughout the biopharma value chain and help identify the optimal strategic approach that moves the needle. Our process ensures that you avoid bias as much as possible, and get through all the IT security, and legal and regulatory hurdles for implementing strategic AI in pharma that creates organizational impact. We also identify optimal vendors and are vendor-agnostic and platform-agnostic with a focus on ensuring you get the best solution to solve your specific strategic challenges. If you have a challenge and you believe there may be a way to solve it with AR but are not sure how, contact us for a strategic assessment.
See more about what we do in this area here.
For more information, contact Dr Andree Bates abates@eularis.com.
Contact Dr Bates on Linkedin here.
Listen to the AI for Pharma Growth Podcast on
For more information, contact Dr Andree Bates abates@eularis.com.