Historically, controlled clinical trials have the primary source of evidence for drug developers and manufacturers. And there’s no denying their importance in the pharmaceutical scientific method. But clinical trials are only a part of the picture when it comes to understanding outcomes .The other part of that picture comes from Real World Evidence (RWE)—data collected from patients and practitioners beyond the controlled setting of a drug trial.
Until recently, however, important technical issues have made it difficult to organise, analyse and draw insights from RWE in a meaningful way. Electronic Health Records (EHRs), for example, are what’s known as ‘unstructured’ data: they lack a standardised format and are written in plain language, as opposed to numbers or boolean expressions like “true” and “false”, which can be easily interpreted by a traditional computer.
Advances in artificial intelligence (AI), and especially in natural language processing (NLP) and machine or deep learning (ML/DL), however, promise to bring these two halves together for a fuller understanding of pharmaceuticals.
In this article, I explore the opportunities that AI offers for bringing RWE more fully into the pharmaceutical fold, as well as some of the technical and structural challenges we still face.
Data, data everywhere…
When it comes to integrating RWE into the pharmaceutical value chain, the biggest problem isn’t that the data doesn’t exist. On the contrary, when we speak of Real World Evidence and Real World Data (RWD), it’s most often in reference to EHRs: notes made in the patient files of hospitals and clinics around the world, of which there is no shortage. Millions of such documents are created by hospitals and clinics every month in the US alone. Indeed, they already constitute an important source of information for outcomes research.
The main problem, though, is that the data is difficult to work with on any kind of very large scale. It is written out in plain language, sometimes even by hand, and structured in a way that’s difficult to aggregate. Efforts to standardise EHRs have had some success, but practitioners aren’t necessarily thinking about data structure when compiling patient notes. Nor, arguably, should they have to; practitioners ought to be able to record information in a simple and familiar way that’s meaningful for their practice.
In addition, practitioners in different regions must comply with different regulatory requirements and operate within the framework of different clinical codes (ICD-9 codes in the United States, for example).
Finally, even within regions and hospital networks, the sheer number of EHR vendors in use is staggering. The average hospital works with 16 different EMR vendors at affiliated practices, which means that practices even within the same hospital network cannot be standardised and easily codified.
Many of these same problems apply to other sources of RWE, such as national health surveys and claims data.
Number of Electronic Medical Record vendors per hospital-affiliated practice.
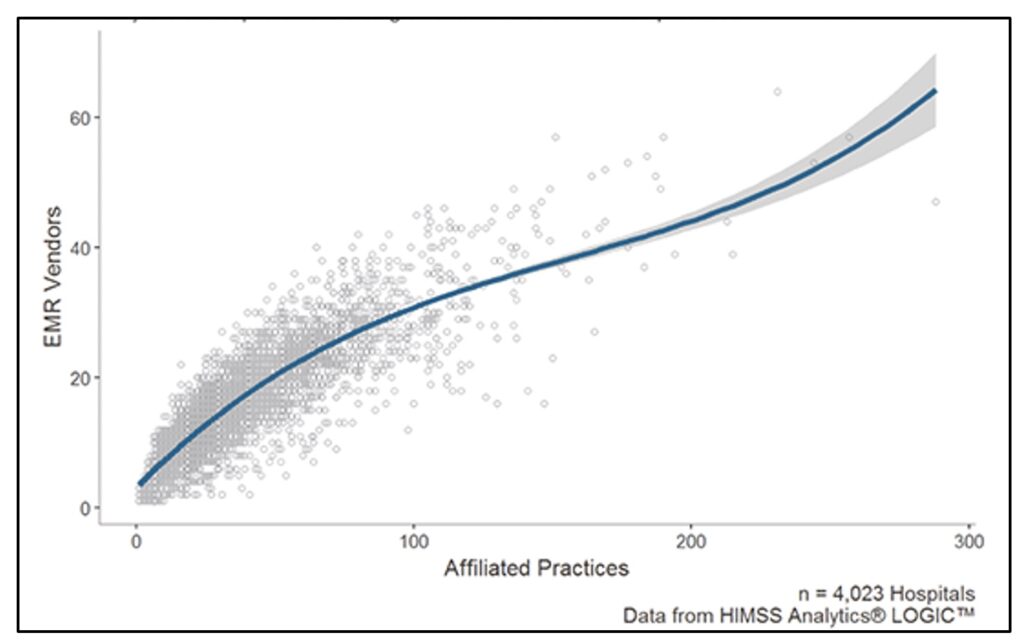
Credit: Healthcare IT News.
There is, therefore, no shortage of real-world data “out there.” It’s just incredibly difficult to standardise, organise, analyse, and interpret.
Or, at least, it was…
Unlocking the potential of real-world data
Advances in AI, NLP and ML have started to change all that. Natural language processing allows computers to pour through millions of plain-language documents, like those written by practitioners and clinicians, and transform the information into data that can be more readily organised and interpreted by both machines and humans.
For example, it is now fairly straightforward to create an NLP solution for pharmaceutical companies that enables them to extract adverse event information from unstructured text inputs and code it into MedDRA data. Once identified and structured, the data can be used in downstream analysis for a host of data-driven decisions.
Importantly, once the data has been thus organised, it can be fed into machine or deep learning algorithms to find deeply hidden patterns and generate important insights, beneficial both to business models and patient outcomes. Provided with enough data of sufficient quality, machine learning algorithms can greatly outperform their human counterparts in, for example, adverse event detection, disease detection, identifying drug interactions, and more.
In a nutshell, NLP offers a way to extract data locked inside real-world data sources like EMRs, and ML can make sense of that data and identify important patterns for a truly data-driven approach to healthcare.
The role of RWE in modern pharmaceutical paradigms
This increased use of real-world data in the pharmaceutical industry not only benefits drug companies, it fits well into a number of important modern-day healthcare paradigms.
1. Outcome-based pricing. This style of pricing sees drug prices set based on patient outcomes. If a drug performs particularly well in the general or a given population, pharmaceutical companies are offered greater returns. If it performs poorly, payments are significantly reduced. In theory, this would incentivize drug companies to focus on patient outcomes as a pricing metric. It is seen by many as one of the most promising opportunities available today for reshaping the industry into one whose value works for patients first.
Gathering evidence of outcomes, however, is tricky business. As much as 70% of the information gathered by outcome researchers comes in the form of unstructured, real-world data, like that mentioned above. Thus, if outcome-based pricing has any chance of succeeding, it must be accompanied by scientific methods that make large-scale outcome research possible, like those mentioned above.
2. Patient-centric approaches. In a similar vein, more and more pharmaceutical companies are taking a patient-centric approach to combat everyday challenges in R&D (like an inability to find eligible trial candidates and patient drop-out), delays in diagnosis, issues with patient adherence, and waning public trust and opinion.
Patient services are now considered critical to positive business outcomes, and an important part of developing and improving those services includes understanding the patient’s real-world experience, their personal and medical history, and other information captured in non-clinical settings. Investing in access and analysis of real-world data will be key for drug companies hoping to take this approach.
3. Owning a disease. “Owning” a disease refers to the practice of using beyond-the-pill approaches like smartphone apps and connected devices to improve patient adherence and outcomes. In doing so, patients-as-customers are provided with a more cohesive and holistic experience, while drug companies gain access to a rich dataset describing individual patients’ healthcare journeys.
To fully own a disease, though, clinical and real-world data must be brought together in a meaningful way. A smart insulin pen can collect precise medical information, but that information only reaches its full value when placed in the context of real-world data like practitioner notes, national health studies, and adverse event reporting.
Challenges at the intersection of AI and RWE:
Access to all this real-world data is an exciting prospect, but like all first forays into new territory, there are important challenges to be addressed.
Data quality and representativity is an important issue when it comes to artificial intelligence in general, and the stakes couldn’t be higher than in healthcare. Machine learning has a tendency to exaggerate and exacerbate biases in data, sometimes with alarming results. Racial, gender and other biases seep into data in insidious and unexpected ways. Data quality also varies significantly between regions, hospital networks, and practitioners. Machine learning algorithms can fill in some of the holes, but new practices, policies and regulations will be required to ensure pharma’s powerful new machine tools are serving patients well.
The questions of data ownership and privacy are at the fore of most discussions around Big Data and artificial intelligence these days. Health records have always enjoyed a special status in this regard, but the proliferation of wearables and industry-adjacent disruptors have blurred these lines. As more and more data becomes available to pharmaceutical companies, issues of de-identification and handling must be addressed.
Historically, data ownership laws favoured private enterprises; only recently, with initiatives like the GDPR and CDPA, has that started to change. Patients have the right to own their data and decide who has access to it when and for what reasons; integrating these rights into the pharmaceutical data pipeline is a necessary step.
Finally, the very fact that real-world data, like EHRs and study results, is so incompatible in its current format with the needs of the pharmaceutical industry points to an important structural divide in the healthcare industry. Sectors as fundamentally related in concept as epidemiology and drug development suffer in practice from a lack of collaboration and information exchange. Making the most of RWE will require greater unity between researchers, payers, practitioners, and pharmaceutical companies
Conclusion
Real-world evidence is an essential but hitherto underutilised resource for data-driven healthcare and positive outcomes for both patients and drug companies. With natural language processing and machine learning, AI promises to bring this data into the pharmaceutical fold.
While challenges still exist, notably around data bias and ownership and collaboration between healthcare players, pharmaceutical companies looking to dramatically improve their operations and successful weather the coming paradigm shifts must champion the integration of RWE into business operations, and AI is the perfect tool to do so.
Found this article interesting?
If you are looking for more insights into how to get access to Real World Data to create Real World Evidence, or to analyze the data you have, contact us to discuss what you need.
At Eularis, we are here to ensure that AI and FutureTech underpins your pharma success in the way you anticipate it can, helping you achieve AI and FutureTech maturation and embedding it within your organisational DNA.
If you need help to leverage AI to identify how to leverage generative AI into your leadership plan to increase operational efficiencies and speed up revenue growth, then contact us to find out more.
We are also the leaders in creating future-proof strategic AI blueprints for pharma and can guide you on your journey to creating real impact and success with AI and FutureTech in your discovery, R&D and throughout the biopharma value chain and help identify the optimal strategic approach that moves the needle. Our process ensures that you avoid bias as much as possible, and get through all the IT security, and legal and regulatory hurdles for implementing strategic AI in pharma that creates organizational impact. We also identify optimal vendors and are vendor-agnostic and platform-agnostic with a focus on ensuring you get the best solution to solve your specific strategic challenges. If you have a challenge and you believe there may be a way to solve it with AR but are not sure how, contact us for a strategic assessment.
See more about what we do in this area here.
Contact Dr Bates on Linkedin here.
Listen to the AI for Pharma Growth Podcast on
For more information, contact Dr Andree Bates abates@eularis.com.